Depression is incredibly difficult to diagnose, but A.I. could help us
Despite being a common disorder, it's still the most difficult to diagnose.
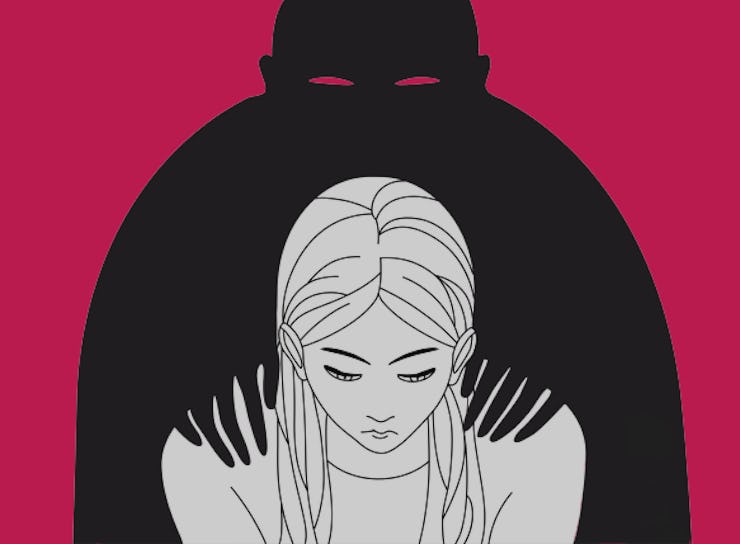
Despite being one of the most common mental disorders, depression is still not well-understood in both research and clinical practice settings. Not all patients present with the same symptoms, which can make it a difficult illness to diagnose. While scientists are hopeful that artificial intelligence can make some order out of the jumble of subjective criteria used to diagnose and treat depression, to date, computational studies still have limitations that have held up the application of machine learning methods in the clinic.
This story originally appeared on Massive Science, an editorial partner site that publishes science stories by scientists. Subscribe to their newsletter to get even more science sent straight to you.
“Diagnostic heterogeneity,” meaning the broad, non-specific symptoms patients present, has been a long-standing criticism of the American Psychiatric Association’s diagnostic tool, the DSM-V, as well as the various scales used to measure depression severity. For example, the DSM-V allows for a high degree of symptom overlap across multiple disorders, which means that a certain combination of symptoms could be diagnosed as two different disorders, a situation clinicians call comorbidity. However, two individuals could also share the same diagnosis with little — if any — symptom overlap. This raises concerns about the validity of saying they have the same condition, especially since finding the right treatment for an individual with depression is done on a trial-and-error basis that can take months.
In psychiatric research, machine learning algorithms are being used to better define depression and to make predictions about which patients might respond to a given treatment. By “mining” data from larger datasets, researchers have been trying to find biomarkers — measurable biological indications — of depression. The thought is that researchers could teach a computer how to identify patterns in data from patient-reported surveys, demographic data, cognitive assessments, and even neuroimaging studies correlating blood oxygenation levels to brain activity in specific regions.
To do this, scientists first input a subset of patient data and adjust their algorithm to reliably distinguish healthy versus control subjects or, in the case of treatment outcomes, responders from non-responders. They can then figure out which features in the data best help the computer “learn,” make sure that their algorithm only incorporates those data features, and validate their method by testing how accurately it can make predictions about the rest of the patients, whose data it has not yet taken into account.
"An inadequate understanding of statistics or software errors can overestimate the predictive power of an algorithm."
This approach has yielded some promising results. Several neuroimaging studies have claimed to have found subtypes of depression. Most recently, scientists from the Okinawa Institute of Science and Technology Graduate University showed that they could identify depression subtypes based on the regions in the brain that have altered activity levels in depressed patients (compared to healthy subjects), combined with the patients’ scores on a survey called the Childhood Abuse and Trauma Scale. Other studies have attempted to tease apart the qualities in patients who respond to treatment with antidepressants or cognitive behavioral therapy.
However, like any lab experiment, computational studies can be poorly designed, limiting the credibility of their findings. As scientists led by Russell Poldrack from Stanford University argue, many studies have too few patients to confidently see an effect in the data, and replication studies have been few and far between. This can magnify other problems with machine learning algorithms. For example, scientists do not necessarily have standardized software packages for these computational studies or even standard methods for processing and analyzing images (in the case of neuroimaging studies). This leaves room for intentional research misconduct, an inadequate understanding of statistics, or software errors to drive a study’s results, leading to misleading conclusions. These mistakes can also overestimate the predictive power of an algorithm. Now with conversations of reproducibility happening more openly, more researchers are pre-registering their studies as well as publishing their datasets and code to address these concerns.
"It is not clear yet whether the patient cohorts … are representative enough for results to be broadly applicable to all depressed individuals."
Researchers have other factors to consider when it comes to data from patient surveys and demographics. While easier and less costly to administer in the clinic than a brain scan, patient-reported data assumes that the patient is reliably reporting symptoms and their severity. Socio-demographic factors also need to be carefully considered to avoid bias. For example, how does the finding from researchers at Yale University that race, education levels, and employment status are among the top predictors of remission of depressive symptoms following antidepressant treatment really help patients?
Additionally, it is no secret that individuals experience depression differently, so regardless of the data source, it is not clear yet whether the patient cohorts included in all of these computational studies are representative enough for results to be broadly applicable to all depressed individuals.
Until these bigger issues surrounding reproducibility and feasibility get sorted out, artificial intelligence will likely not make psychiatric evaluation less subjective.