To predict Covid-19 mutations, scientists discover one fifth-grade tactic is surprisingly useful
And no, it's not wedgies or shame.
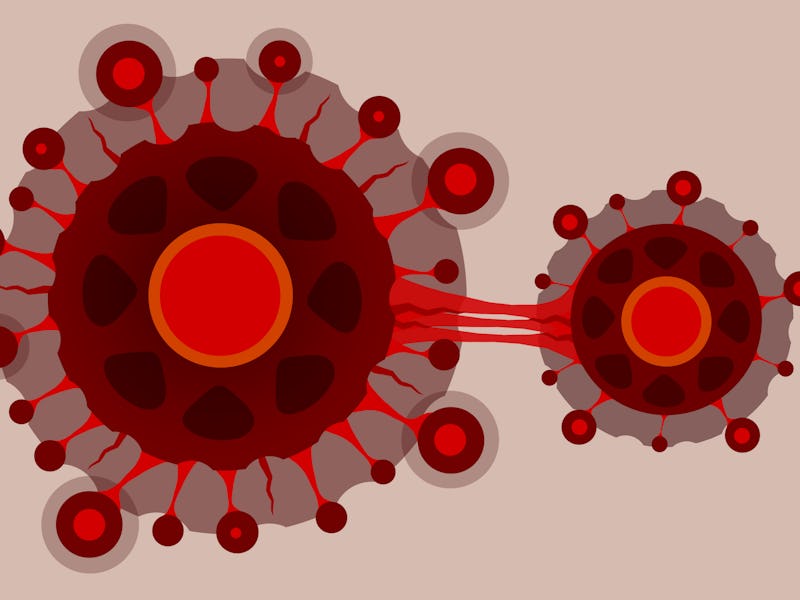
Dissecting a sentence's grammar is a hobby typically reserved for elementary school teachers or that pedantic English major you met in college, but a team of scientists from MIT is interested in whether these same linguistic methods can actually tell us something about emerging Covid-19 mutations.
In a new paper, published Thursday in the journal Science, a team of computer scientists and biological engineers report striking parallels between how we parse sentences for grammar and syntax and how virus proteins morph and "escape" the body's immune system.
By creating a machine-learning algorithm based on this idea, the team was able to predict potential mutations on the horizon for different viruses, including HIV and SARS-CoV-2.
Why it matters — Three of the study's authors, Brian Hie, Bonnie Berger, and Bryan Bryson spoke with Inverse about this finding. They tell us their method could help "rapidly flag" new Covid-19 mutations, enabling scientists to quickly evaluate the threat they pose, and could even unravel the evolutionary origins of such mutations.
Here's the background — When it comes to teaching computers to recognize language patterns, the researchers didn't have to start from scratch. Teaching machines the basics of language is a question that goes all the way back to Alan Turing's work in the 1950s, and has since launched an area of study called "natural language processing" (NLP.)
Natural language processing used in computer science may be key to tracking down emerging virus mutations.
Similar to how children learn their native language by immersion, NLP algorithms learn about language by being fed reams and reams of written text. These algorithms can pull out patterns and can patch words together to form (sometimes) meaningful sentences — it is essentially the same technology that powers the autocomplete on gmail, for example.
What the researchers behind the new study realized is that the same method could be applied to other information — like the combination of amino acids in a virus.
"We were excited about recent advances in NLP language models for understanding human language by training them on raw text alone," the study authors tell Inverse. "We thought that since the most abundant data for viruses is just raw viral sequence, we could also learn very complex patterns from viral sequence datasets by training a language model."
Digging into the details — Essentially, instead of training an NLP model on language from books, the team theorized that they could train it on raw virus data to find similar patterns. In particular, they were interested in how methods used to identify changes in grammar and syntax in any given language over time could be used to estimate "viral escape" — the threshold at which a virus mutation changes the virus enough to fool the immune system, but not so much that it becomes less "fit" or effective at infecting hosts.
This video shows the Covid-19 virus' spike protein and how it might evolve to reach the point of "escape."
"What we show in the paper is that to escape the immune system, a virus needs to preserve grammaticality — i.e., follow a set of biological rules — while altering its semantics — i.e., changing itself enough to look different to the immune system," the authors explain to Inverse.
To understand, let's go back to language. Take, for example, the sentence: "The boy pats the dog."
One change (or mutation) you could make would be to write "the boy pets the dog." This change maintains the original's grammar and semantics (i.e. what the sentence means). But if you were to instead write "the boy eats the dog," then you'd have a big enough semantic change to change the meaning, but still preserve the grammar. It's this kind of change that the team was looking for in the viruses.
While previous research had tried similar approaches, focusing either on semantic change or fitness change through "grammar", the researchers say they are the first to combine both in a single model.
What they discovered — With their language model trained up on amino acids, the researchers then tested its ability to predict likely mutations of viruses including influenza, HIV-1, and SARS-CoV-2 — viruses notorious for their ability to dodge universal vaccines by mutating (SARS-CoV-2 being the exception — we don't yet know if the virus can mutate enough to circumvent a vaccine).
Using computer models, the team created all possible single-strain mutations of the given virus protein sequences and tasked their algorithm with objectively ranking their likelihood to reach viral escape. Comparing the estimates made by their dual grammar-semantics approach, the researchers report this model was better at accurately predicting these mutations than just grammar or semantics alone. In fact, if either parameter was ignored, accuracy went down.
"Our analysis suggests a way to quantify the escape potential of interesting combinatorial sequence changes, such as those from possible reinfection," the authors write in the paper.
The team's language processing model assigned color coding to the virus models to identify proteins with more likelihood of mutating.
What's next — While these initial results are promising, the authors tell Inverse there are significant limitations to their current model they need to overcome.
"The limitations are that right now, we assume semantic change corresponds to changes that a virus makes to evade the immune system," the team tells Inverse. "In reality, while immune evasion is one component of semantic change, there could be other kinds of natural selection also embedded within semantic change — for example, semantic change in response to drug selection."
"So we are really excited about investigating other kinds of evolutionary pressure in the future and potentially separating different kinds of pressure from each other," they add.
A separate limitation is that the system is trained on existing virus data, meaning it may perform differently as new data comes in and scientists become aware of new viral strains.
But for right now, the researchers say they're excited about the role this model could play in flagging and addressing new Covid-19 mutations, making the emergence of new variants like B.1.1.7 — the variant currently blitzing its way through the United Kingdom and elsewhere — much more manageable.
"We think our model can rapidly flag new sequences that are substantially different from previously seen viral sequences for further testing in the laboratory. You could imagine each new sequence gets examined by our model and sequences that are altered beyond a certain threshold need to be looked at in the lab for further study," they say.
Abstract: The ability for viruses to mutate and evade the human immune system and cause infection, called viral escape, remains an obstacle to antiviral and vaccine development. Understanding the complex rules that govern escape could inform therapeutic design. We modeled viral escape with machine learning algorithms originally developed for human natural language. We identified escape mutations as those that preserve viral infectivity but cause a virus to look different to the immune system, akin to word changes that preserve a sentence’s grammaticality but change its meaning. With this approach, language models of influenza hemagglutinin, HIV-1 envelope glycoprotein (HIV Env), and severe acute respiratory syndrome coronavirus 2 (SARS-CoV-2) Spike viral proteins can accurately predict structural escape patterns using sequence data alone. Our study represents a promising conceptual bridge between natural language and viral evolution.
This article was originally published on