This wearable could help you avoid shin splints forever
The runner's bane could be a thing of the past.
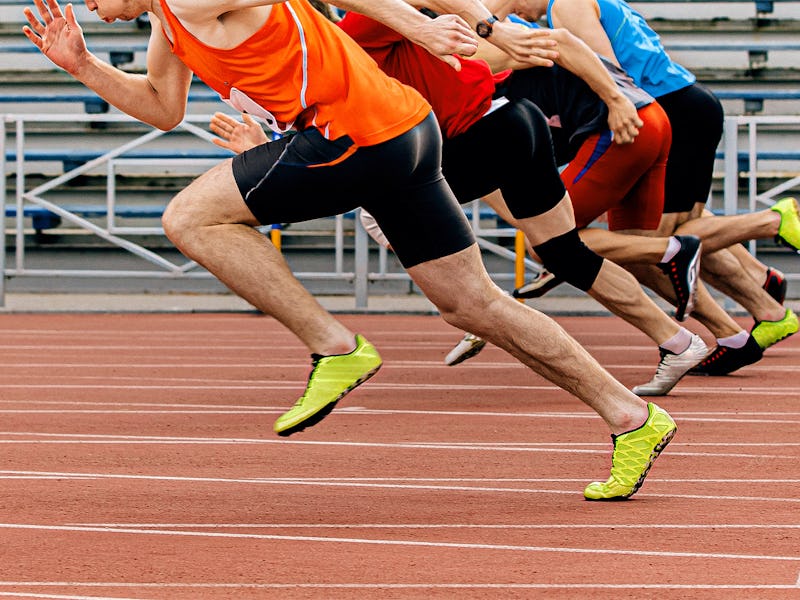
They might start as a dull ache on mile two of the morning jog, or erupt as a spasm of pain when you lunge to return your opponent's serve — with every step you take, you feel the ground strike back with equal force.
Shin splints are a well-known, universally unwelcome reality for amateur and professional athletes alike. This aching pain commonly appears during exercise and is caused by stress on your tibia (aka, shin) bone and surrounding muscle.
Two weeks of rest is usually enough to heal the strained tissue, but if exacerbated, shin splints can result in more serious stress fractures.
Sport medicine doctors take an invasive approach to monitoring tibia stress in athletes, including implanting monitors directly in tissue. Away from the clinic, amateurs are left to struggle on — but their troubles could soon be over.
New research, led by Vanderbilt assistant professor of mechanical and biomedical engineering and physical medicine & rehabilitation Karl Zelik, proposes taking an innovative and practical approach to measuring this internal stress that could help runners better monitor and even prevent shin splints. The findings were recently published in the journal Human Movement Science.
Emily Matijevich, a biomechanics Ph.D. student at Vanderbilt and first author on the study, tells Inverse that helping runners deal with shin splints and other bone stress may not only help prevent physical pain, but psychological pain as well.
"[Shin splints are] painful physically but it also has psychological impacts as well," explains Matijevich. "Getting sidelined for two or three months during [a sports] season can be distressing."
The initial inspiration for the team's device came when a sports medicine doctor came to them to commiserate about the number of tibial stress injuries that were coming in and out of this clinic every week, Zelik says.
"This was a project that we didn't plan to take on," he explains.
"But a sports medicine doctor approached us about four years ago now and had mentioned to us that he has runners showing up in his clinic week in and week out with stress fractures," Zelik tells Inverse.
"He was really interested in whether he could use wearable sensor technology to help monitor these runners and get them to stop showing up at his clinic."
Zelik and the team have been trying to find an answer ever since.
The team published some initial findings in the journal PLOS One in 2019. In that paper, they found that the commercially available wearables claiming to measure (or at least, estimate) tibial stress were no better equipped to do so than a simple pedometer.
The problem was that the wearables measured the wrong sensor signal altogether when making these assessments. Because fitness wearables like watches or pressurized insoles can't actually access the tissue around your shins to measure stress, they instead rely on something called 'ground reaction force' (e.g. how hard you land on the ground with each step) to estimate potential stress.
"The thought of your foot hitting the ground and there being that impact and interaction force intuitively, makes you think that perhaps that's what is causing wear and tear on your body," Matijevich explains.
"But while we have that sensory feedback of our foot impacting the ground, actually the peak forces on our bone happen midway through our running stride, so not really near that initial contact."
The researchers found that existing tibial stress algorithms were placing their bets on the wrong kind of sensor data.
Worse yet, for every degree of error in the under or overestimation of stress, the potentially negative affects on the bones increase exponentially, she says.
With this knowledge in hand, the team set out to improve these stress estimations using existing sensors and wearables. The team used data from ten runners across thirty different running conditions to see how both a physics-based algorithm and a machine learning-based algorithm would fare in predicting actual tibial stress. To make these predictions, the algorithms incorporated multi-sensor data measuring speed, slope, pressure, and angle of the foot and leg.
The physics-model made predictions based on an individual runner's biomechanics. By contrast, the machine learning-based model was trained on the performance and tibial stress measurements of nine of the ten runners, and then tested on the tenth.
The team then compared their estimations to those of existing ground force reaction-based algorithms to see which was most accurate. The existing algorithms had an average error rate between 8 - 11 percent, while the team's physics-based and machine-learning algorithms show only a 5 and 3 percent error rate, respectively.
Here's where the research gets exciting for runners: The findings suggest the necessary improvements can be made to existing wearable hardware with just a simple software upgrade, Zelik says. The approach can also be applied to other areas of the body, like the lower back, he adds.
The fact it could be so simple as upgrading current wearables means bone stress monitors could come in many forms — from pressure-sensing insoles, to shoe or wrist wearables. Everyone from professional athletes, weekend warriors, and even sport medicine doctors could stand to benefit, Zelik says.
See also: A startling running stat proves more isn't always better
The Vanderbilt team are in talks with the sports industry to commercialize their product. Runners can expect to see wearables like this on the market within the next five years, or even sooner, they predict.
So, is this the end of shin splints as we know and loathe them? Depending on how religiously you use the sensor, Zelik says it's possible to greatly reduce the prevalence of this common injury.
"I think by measuring these [injuries] and understanding when people are at risk you can absolutely have a positive impact on you know how much risk people are exposed to and as a result, over time, reduce the number of injuries," Zelik says.
Abstract: There are tremendous opportunities to advance science, clinical care, sports performance, and societal health if we are able to develop tools for monitoring musculoskeletal loading (e.g., forces on bones or muscles) outside the lab. While wearable sensors enable non-invasive monitoring of human movement in applied situations, current commercial wearables do not estimate tissue-level loading on structures inside the body. Here we explore the feasibility of using wearable sensors to estimate tibial bone force during running. First, we used lab-based data and musculoskeletal modeling to estimate tibial force for ten participants running across a range of speeds and slopes. Next, we converted lab-based data to signals feasibly measured with wearables (inertial measurement units on the foot and shank, and a pressure-insole) and used these data to develop two multi-sensor algorithms for estimating peak tibial force: one physics-based and one machine learning. Additionally, to reflect current running wearables that utilize foot impact metrics to infer musculoskeletal loading or injury risk, we estimated tibial force using the ground reaction force vertical average loading rate (VALR). Using VALR to estimate peak tibial force resulted in a mean absolute percent error of 9.9%, which was no more accurate than a theoretical step counter that assumed the same peak force for every running step. Our physics-based algorithm reduced error to 5.2%, and our machine learning algorithm reduced error to 2.6%. Further, to gain insights into how force estimation accuracy relates to overuse injury risk, we computed bone damage expected due to peak force. We found that modest errors in tibial force translated into large errors in bone damage estimates. For example, a 9.9% error in tibial force using VALR translated into 104% error in bone damage estimates. Encouragingly, the physics-based and machine learning algorithms reduced damage errors to 41% and 18%, respectively. This study highlights the exciting potential to combine wearables, musculoskeletal biomechanics and machine learning to develop more accurate tools for monitoring musculoskeletal loading in applied situations.