A next-gen safety algorithm may be the future of self-driving cars
Hopefully this will put an end to self-driving collisions.
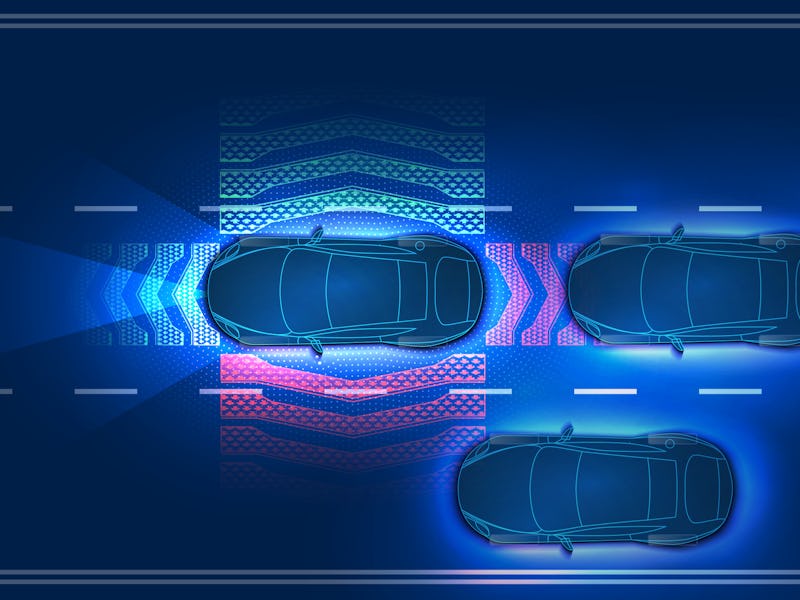
Taking a snooze at your car's steering wheel as it hurdles down a freeway is a terrible idea, but with the continued expansion of self-driving car technology, getting a few extra minutes of sleep on your commute may not remain a pipe dream for much longer.
But there are more than a few issues with this vision of the future. Chief among them is that the people don't trust self-driving cars, at least not enough to confidently put their lives in vehicles' "hands."
Research from computer scientists in Germany could swing public opinion, however. Armed with a new algorithm, autonomous vehicles would be able to make safety calls in real-time. The idea is that the vehicles would only be able to make harm-free choices, protecting passengers and pedestrians alike.
Establishing trust in self-driving vehicles is an essential step toward getting more self-driving cars on the road — and ultimately driving down vehicle fatalities overall.
In a study published Monday in the journal Nature Machine Intelligence, the researchers explain how their approach differs from typical probabilistic machine learning algorithms in a few key ways, Christian Pek, the study's first author and a post-doctoral researcher at the Technical University of Munich (TUM) at the time of the research, tells Inverse.
"A probabilistic analysis usually generates a model that tries to mimic the distribution of what's probably going to happen next," Pek explains. This could lead to a collision if that model's view of the world (like predicting an oncoming car will probably turn right) disagrees with reality (like it actually turns left and they collide).
And because the decision-making process of a machine learning algorithm is typically a black box, meaning it's hard to always see their "thought process," it can be difficult to know if "an unsafe decision was taken," Stefanie Manzinger, a co-author on the study and doctoral candidate at TUM explains to Inverse.
To get around the issue of unpredictability, the researchers took a "reachability-based" approach, meaning their model is designed only to look at feasible solutions that satisfy a set of baked-in traffic and safety rules. The rules used by this algorithm are based on guidelines for human drivers from the Vienna Convention of Road Traffic.
They boil down to simply "avoid[ing] any behavior likely to endanger or obstruct traffic," the paper reads. This is, assuming all other vehicles and pedestrians follow the rules, too. The researchers call these guidelines "legal safety."
Unlike a probability model, Pek says that this reachability model can consider all legal and physically possible traffic moves at once and find a safe trajectory for the vehicle.
"You're 100 percent safe against legal safety," says Pek.
But, that doesn't necessarily mean the algorithm can protect you against all forms of danger on the roads. Malicious driving (like purposefully driving into traffic,) for example, is not protected against by this algorithm because it is not considered a legal form of driving.
This algorithm is designed to anticipate and play out all possible scenarios before suggesting a next move to a self-driving vehicle.
How does it work — Training a car not to swerve into traffic may sound simple on the face of it, but when hard-to-predict variables like human drivers or pedestrians are factored in, this can result in infinite real-life scenarios.
Instead of training vehicles to memorize millions of different traffic scenarios, the algorithm analyzes different players' trajectory during an unexpected event, and then calculates the safest route possible given different environmental variables, like the vehicle's speed, and surrounding obstacles like pedestrians.
The algorithm is designed to make these decisions in real-time, essentially letting the vehicle's controlling computer "freeze" time to explore all the different realities each event poses. Then, the computer creates a path forward that would avoid all the potential adverse realities in all possible timelines.
Or, if there is no safe trajectory forward, the algorithm defaults to a fail-safe, which safely removes the vehicle from traffic.
How they tested it — This study did not test the algorithm in a true traffic scenario, but they did test it on the next best thing — real traffic data. Instead of using simulated traffic scenarios which may not account for the true variability on the road, the researchers used real traffic videos and other data, and applied their algorithm.
When applying the algorithm to different, real-life scenarios — like turning left at a busy intersection or avoiding a jaywalking pedestrian — the researchers found that their algorithm suggested a collision-free trajectory every time.
What's next — Replacing human drivers with fleets of smart, self-driving cars is predicted to result in a whopping 90 percent reduction in traffic-related fatalities. Based on 2017 data from the U.S. National Highway Traffic Safety Administration, that could save nearly 30,000 lives a year. The researchers behind this study hope that their algorithm can play an essential role in building public trust in these vehicles and moving towards this safer future.
"To achieve widespread acceptance [of self-driving vehicles,] safety concerns must be resolved to the full satisfaction of all road users," write the authors.
"[In this work] we call for a paradigm shift from accepting residual collision risks to ensuring safety through formal verification...As a result, we expect that societal trust in autonomous vehicles will increase."
Abstract: Ensuring that autonomous vehicles do not cause accidents remains a challenge. We present a formal verification technique for guaranteeing legal safety in arbitrary urban traffic situations. Legal safety means that autonomous vehicles never cause accidents although other traffic participants are allowed to perform any behaviour in accordance with traffic rules. Our technique serves as a safety layer for existing motion planning frameworks that provide intended trajectories for autonomous vehicles. We verify whether intended trajectories comply with legal safety and provide fallback solutions in safety-critical situations. The benefits of our verification technique are demonstrated in critical urban scenarios, which have been recorded in real traffic. The autonomous vehicle executed only safe trajectories, even when using an intended trajectory planner that was not aware of other traffic participants. Our results indicate that our online verification technique can drastically reduce the number of traffic accidents.