Robots and humans can work together to master an ancient sport
Curly the robot has defeated top curling teams. But the sport could offer a prime opportunity to bring people and robots together.
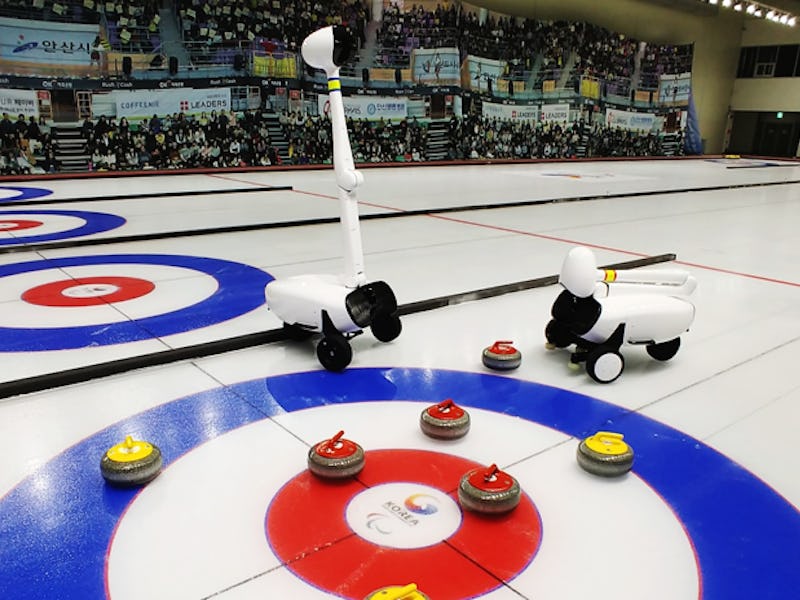
When the United States won the gold in curling at the 2018 Winter Olympics, many Americans responded to the news with a simple question: What is curling?
But despite the ignorance of the sport among some humans, robots are learning the rules — and beating the best of our human teams at their own game, too.
In a new study published Wednesday in Science Robotics, researchers describe how a robot named Curly defeated human teams in the sport, three matches to one. But rather than feel inferior to our artificially intelligent competitors, take heart — Curly is also an example of how humans and machines might one day work together.
Curly, right after throwing a stone.
Seong-Whan Lee is a study author and professor at Korea University. He tells Inverse curling is a perfect testing ground for artificial intelligence.
That is because curling is a “combination of bowling and chess; it is a turn-based game in which two teams play alternately on the ice sheet, requiring a high level of strategic thinking and performance," he says.
Curling requires a mixture of tactical planning and delicate physical touch, two areas where artificial intelligence needs to improve. “The environmental characteristics change at every moment, and every throw has an impact on the outcome of the match," Lee says. That means teams need to change their strategy and adapt on the fly.
Because of the subtle changes that take place after every throw, the team behind Curly used a deep reinforcement learning system, a type of AI that learns through trial and error. After learning the game via many simulations, Curly’s stones had indoor GPS sensors attached to them.
Taking stock of the game’s situation after every throw, Curly would analyze where to throw next. Crucially, Curly did not have to relearn how to throw after every attempt. Rather, the AI could re-calibrate itself, allowing it to respond to the new situation.
Curling teams traditionally have three main player types: a thrower, a skip, and sweepers. The thrower's job is obvious, while a skip is equivalent to a field general or coach, directing throwers on best strategies from the other side of the rink. Sweepers provide the sport’s most memorable visuals, using brooms to sweep away at ice in front of the stone to direct its motion.
Curly's thrower and skip components discussing strategy.
All of that manpower is hard for a robot to replicate: Curly’s throwing component is a wheeled robot that spins the stone and releases it. Curly does not yet have a sweeper component, which worked out well enough in its matches against the Korean national wheelchair curling team, as the wheelchair version of the sport does not require sweeping.
To fully understand Curly’s abilities as a player, the bot wasn't limited to playing against humans. Curly also played games with humans, using them as sweepers.
“These special matches are a good example of collaborative robots in the real world. Curling is certainly a very challenging environment, and we found that humans and robots can cooperate very well, as was also observed in previous collaborative robot scenarios,” Lee says.
As exciting as Curly’s adventures on the ice have been — Lee highlights Match D against the Korean national wheelchair curling team as a “close match” — the technology doesn’t only apply to curling.
Curling works as a good example of a situation where there isn't a lot of time to completely recalibrate an AI system, but there are numerous other possibilities. Lee says the trial-and-error style learning helps Curly move on the ice could enable other adaptive tech, like flying drones.
Abstract: The game of curling can be considered a good test bed for studying the interaction between artificial intelligence systems and the real world. In curling, the environmental characteristics change at every moment, and every throw has an impact on the outcome of the match. Furthermore, there is no time for relearning during a curling match due to the timing rules of the game. Here, we report a curling robot that can achieve human-level performance in the game of curling using an adaptive deep reinforcement learning framework. Our proposed adaptation framework extends standard deep reinforcement learning using temporal features, which learn to compensate for the uncertainties and nonstationarities that are an unavoidable part of curling. Our curling robot, Curly, was able to win three of four official matches against expert human teams [top-ranked women’s curling teams and Korea national wheelchair curling team (reserve team)]. These results indicate that the gap between physics-based simulators and the real world can be narrowed.