AI can now detect pancreatic cancer better than radiologists
The technology could help combat one of the hardest-to-detect (and deadliest) diseases.
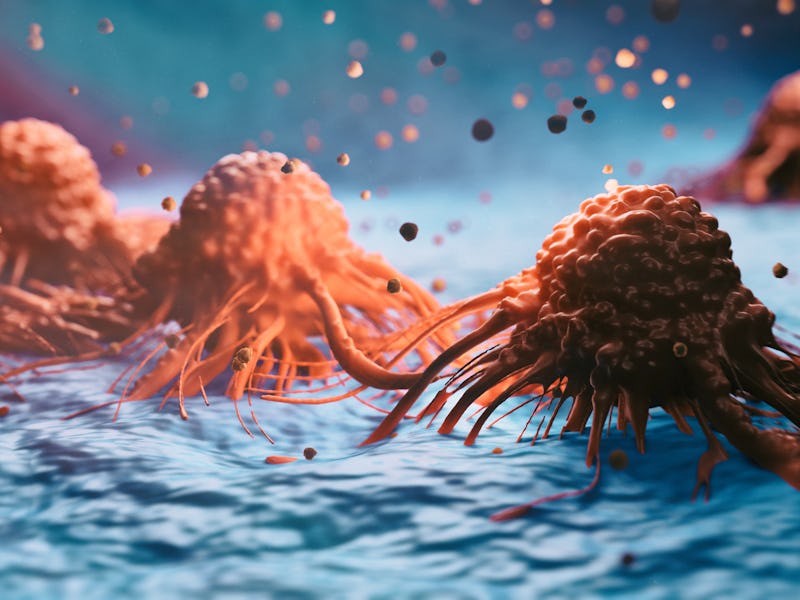
In recent years, AI has infiltrated hospitals: We now have everything from digital nursing assistants to robotic surgeries. For their next feat, computers are taking on cancer detection.
Scientists have developed a new AI system that can spot signs of pancreatic cancer missed by even the best radiologists, according to a new study published in the journal Radiology.
This novel tech, which will likely roll out in Taiwan in the near future, adds to the argument that AI can help improve health care and give doctors and hospital specialists more time with patients.
“I think AI can do two things: the first is to help doctors do what they can, but with less time and energy,” says Wei-Chi Liao, professor of internal medicine at the National Taiwan University and one of the lead study authors. “And the second is to help doctors do what they cannot always do; for example, in our study, detect cancers that are not very visible to humans.”
Here’s the background — Pancreatic cancer is one of the most deadly types of the disease (after lung and colorectal cancers). It will kill over 49,000 people in 2022, according to the American Cancer Society, and only about 20 percent of patients survive a year post-diagnosis.
That’s because the chances of recovery plummet once the tumor grows larger than 2 centimeters, at which point it spreads rapidly and aggressively to other organs.
Pancreatic tumors don’t usually have clear borders with surrounding tissue, and CT scans miss around 40 percent of small masses.
And while early diagnosis is crucial, it’s hard for doctors to spot a pancreatic tumor before it reaches that length. Typically, the nascent stages don’t cause symptoms in patients.
The tricky-to-catch tumor often doesn’t have clear borders with the surrounding tissue, Liao says, and the current imaging techniques using CT scans miss approximately 40 percent of tumors smaller than this size. So patients often miss their only opportunity of treating their condition before it’s too late.
What’s new — But that could change with the National Taiwan University team's new technology, which has outperformed experienced radiologists in testing. It’s essentially a combination of five deep-learning models.
“So it's just like saying we have five experienced radiologists to look at the same case and they seek for their consensus,” says Weichung Wang, director of National Taiwan University’s MeDA Lab and a lead study author.
By feeding the system over hundreds of examples of tumors, the researchers taught the AI to recognize pancreatic cancer with 91 percent accuracy.
What they did — First, they tested their AI system on scans from 1,279 patients — 546 with pancreatic cancer and 733 without — and the tool achieved 90 percent sensitivity, which is a test’s ability to detect if somebody has cancer.
It also reached 96 percent specificity, or its efficacy at sniffing out the absence of cancer. This helps the system avoid false positives.
Scientists sourced data from around Taiwan to make sure their AI system was up to snuff.
To give it a real challenge, the team also tried the system out on retroactive data from real-world patients. After all, testing AI systems outside of the lab can make or break a new technology.
“When you test the model using some outside data, the performance tends to deteriorate quite significantly and there is a significant ‘generalizability problem,’” Wang says.
Why it matters — With data sourced from 1,473 people in hospitals across Taiwan, the AI scored 90 percent sensitivity and 93 percent specificity. When it came to tumors smaller than 2 centimeters, the system spotted them 75 percent of the time.
While 75 percent may not sound very impressive, radiologists only find smaller tumors about 60 percent of the time, Liao notes.
What’s next — The system is currently used in the National Taiwan University Hospital for research purposes, as it hasn’t yet received regulatory approval.
There, doctors and radiologists download the system on hospital iPads and, after uploading CT scan images to a server, the app annotates them with its findings.
It could be deployed across Taiwanese hospitals in less than a year, Liao notes, but it might take longer to be approved internationally. If all goes well, it could make a major difference in the U.S.
“I think these findings are game-changing,” says Linda Chi Hang Chu, professor of radiology at John Hopkins University, who was not involved in the study.
The new AI system could be applied to all abdominal CT scans to catch hidden cases.
She notes that several groups, including her colleagues at Johns Hopkins, hope to harness AI to diagnose pancreatic cancer. Deploying this tech in community hospitals and the locations where patients are first seen could make all the difference in improving the chance of diagnosis at a curable stage, Chu says.
These detection systems should be used to analyze all abdominal CT scans — not just CTs taken to analyze a pancreatic mass.
Patients often arrive at the hospital with “abdominal pain,” which is why radiologists might miss the cancerous mass: They’re not looking specifically for it. AI, on the other hand, could do just that.
But the tool isn’t quite yet ready for prime time, since it needs to be trained on wider and more diverse populations, according to Wang.
“It is very important to generalize the AI, to see how it works in the real world,” he says. “In the real world, that data can be very, very messy, and noisy.”