This incredibly simple A.I. program can decode humans' most mysterious quality
When used in a mouse model researchers identified unique reactions to emotional stimuli.
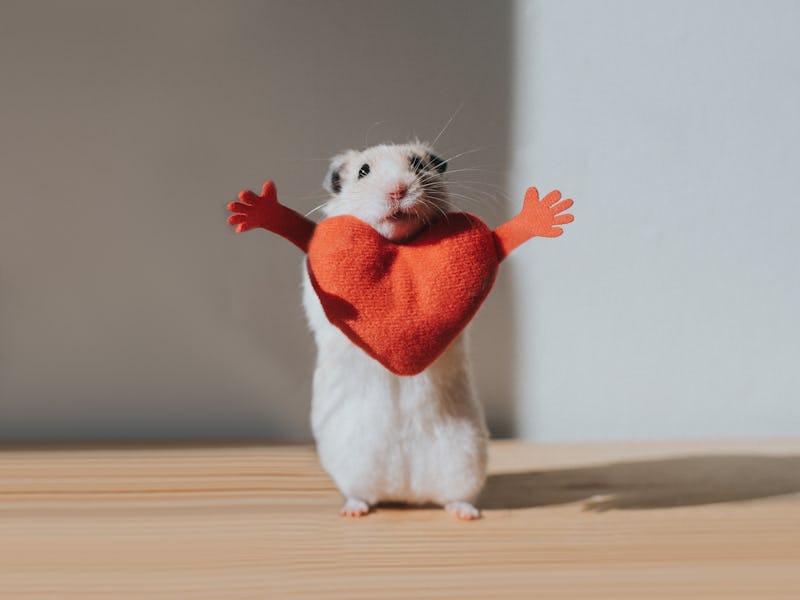
If you've ever broken down crying unexpectedly or laughed during an awful situation, you'll know that human emotions are an incredibly complex and confusing thing. To better understand the origin of these potent emotions, scientists have turned to a group of tiny, emotional mice to crack the code.
Using a mix of machine learning and brain-imaging, a team of researchers was able to map the physical reaction to certain sensory stimuli in mice and see how those responses correlated to the firing of neurons in the brain. The scientists say this brings them even closer to finally understanding the true origin of emotions in our brains.
In the study, published Friday in the journal Science, the authors began exploring these questions by learning how mice would emotionally respond to different sensory stimuli. To see a range of emotional responses, the team exposed the mice to stimuli such as sweet and bitter tastes and painful tail shocks to stimulate emotions like pleasure, disgust or fear. After receiving this stimuli, or "emotional events," the mice reacted with visible facial responses. However, the authors write that human observation of these differences can be tedious and subjective.
Instead, they turned to machine learning to help classify the events, Nadine Gogolla, co-author and researcher at the Max Planck Institute of Neurobiology, tells Inverse.
The algorithm was able to predict with 90 percent accuracy what emotional event the mouse had experienced based only on the facial reaction they made.
"It would have been a lot of work [for] human observers trying to distinguish [between emotions,]" says Gogolla. "But it would have been much less objective and quantitative to do this with human observers... We made it more objective and more quantitative by using this machine vision and machine learning approach."
Using machine vision, scientists were able to quantitatively measure mice's reaction to sensory stimuli.
Feeding their computer vision algorithm a video feed from the emotional events, the researchers found that the mice in their experiment reacted similarly to the different stimuli: during a scary or fearful event, they would lower their ears. These reactions became the norm, and were eventually so stereotyped that the algorithm was able to predict with 90 percent accuracy what emotional event the mouse had experienced based only on the facial reaction they made.
However, this result alone wasn't enough to draw any real conclusions about the underlying emotions the mice were feeling. What if these reactions were simply a physical reflex? To prove these reactions were more than mere reflexes the researchers explored what would happen when they scaled the strength of the stimulus. Using their computer vision algorithm they found that the mice's reactions scaled with the strength of the stimulus, suggesting that it was more than merely a knee-jerk reaction.
To separate social interaction the mouse's emotional response, the scientists created an experimental set-up to film the mouse's reaction while holding it stationary.
Gogolla tells Inverse that they were also able to quantify other traditional emotional attributes in their mice, such as a generalized emotion response and a memory-based emotional response. To test this they first gave a mouse a sweet solution and then traded it for a bitter one in the next round. When that mouse returned again to the sweet solution they reacted in disgust because of their negative memory. Had the sweet solution only been triggering a biological reflex instead of an emotional response, the mouse likely would have reacted with pleasure despite their negative experience, Gogolla explained to Inverse.
The researchers were able to further confirm these results by peeking inside the mice's brain using two-photon calcium imaging and looking for which neurons might be controlling these reactions. Through evaluating how neurons were firing during these emotional events the team identified a number of neurons that could be directly correlated with the mice's different facial expressions.
Using computer vision the researchers transformed mice's emotional reactions into qualitative data.
"We demonstrated that we really now have a precise and also a dynamic way of reading out and measuring emotional states," says Gogolla. "Because if this is truly the case then we should be able to find neuronal correlates to those [emotional states] -- and we did. If they were just more or less a feature of the machine vision, which has nothing to do with the true emotional experience, then it would be very hard to argue that we can find neural correlates in the brain."
This result suggests that different parts of the brain are responsible for encoding distinct emotions, write the authors. While their work is currently based on a mice model, their result sheds light on where the origin of human emotion might lie as well.
"Emotions have traditionally been studied by different fields," says Gogolla. "At the moment, there's no consensus on a definition of what emotions really are... One way we could come to a more qualitative description would be by finding and understanding the brain correlates [as in this study.] Then we can get further and further towards a more biologically based description of emotions and I think in the long term that would help."
Abstract: Understanding the neurobiological underpinnings of emotion relies on objective readouts of the emotional state of an individual, which remains a major challenge especially in animal models. We found that mice exhibit stereotyped facial expressions in response to emotionally salient events, as well as upon targeted manipulations in emotion-relevant neuronal circuits. Facial expressions were classified into distinct categories using machine learning and reflected the changing intrinsic value of the same sensory stimulus encountered under different homeostatic or affective conditions. Facial expressions revealed emotion features such as intensity, valence, and persistence. Two-photon imaging uncovered insular cortical neuron activity that correlated with specific facial expressions and may encode distinct emotions. Facial expressions thus provide a means to infer emotion states and their neuronal correlates in mice.