A.I. 'memory bottleneck' solved with tiny, magnetic material
There's more data being created every day. Too much data. Antiferromagnetic materials could help.
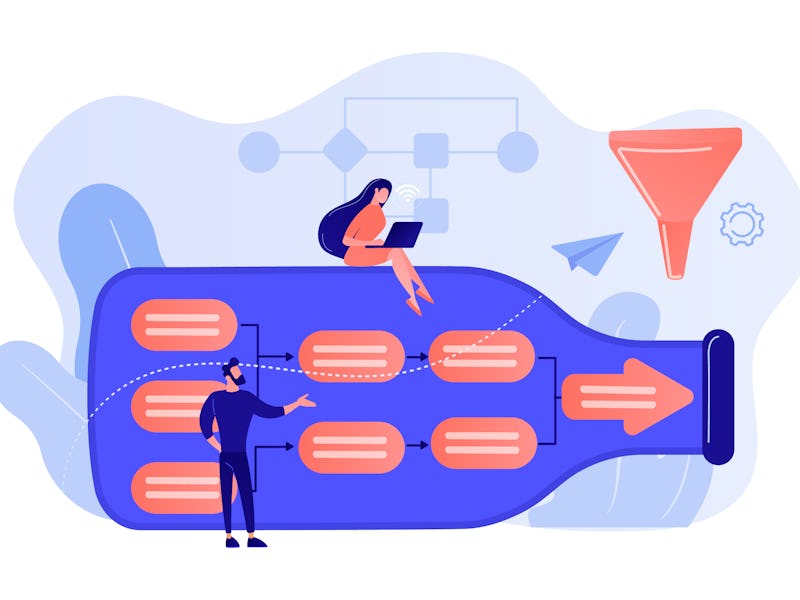
According to a report from the International Data Corporation (IDC) in 2018, we are only five years away from reaching a data saturation of 175 zettabytes. That data could fill enough BluRay discs to reach the moon 23 times and fill 12.5 billion of the world's largest hard-drive if downloaded. Even more concerning, the report says that nearly half of this data -- 87.5 zettabytes -- will be stored in the cloud. This includes every social media platform you use and every remote work document you open. With billions of people trying to access that data all at once, this kind of memory storage has a bottleneck problem.
While computing is getting smarter and smarter, those advances could be moot if hardware can't scale to support its growing trove of data. Typical storage methods can grow slow with data influx or dissipate too much power in the process which can lead to what researchers call a "memory bottleneck." To address this large problem, researchers have turned to tiny magnetic materials.
These materials, called antiferromagnetic materials (AFMs,) are imbued with electrons that behave like tiny magnets and are capable of recording memory with just this magnetism alone without the added boost of an electric current. Because the quantum spin of the material's electrons are antiparallel (parallel but moving in opposite directions) the material does not demonstrate macroscopic magnetism and as a result, is resistant to outside magnetic fields that might potentially erase its data. AFM's storage capabilities, as well as their resistance to tampering, make them attractive options for storing important data.
But these same anti-tampering characteristics make it difficult for researchers to adjust the magnetic state of this material in manufacturing. A study published in the journal Nature Communications attempts to solve this problem by applying electric currents that reveal multilevel memory capabilities in these materials.
To accomplish this, researchers designed AFM pillars with diameters of only 800 nanometers constructed from platinum manganese. These pillars are ten times smaller than previous AFM models. Through experimental trials, the research team was able to apply small electric currents to their AFM model and effectively change the magnetic order of the material. In addition to demonstrating more control of this material's magnetic state than previous research, the authors also write that the heavy metals used in their model are already compatible with memory chip and semiconductor manufacturing practices, meaning it would be relatively easy to translate this approach into a commercial reality.
Northwestern University Associate Professor of Electrical and Computer Engineering and co-lead on the study, Pedram Khalili, said in a statement that their model could help bridge the gap between the computing capabilities of A.I. and its physical limitations.
"The rise of big data has enabled the emergence of artificial intelligence (AI) in the cloud and on edge devices and is fundamentally transforming the computing, networking and data storage industries," said Khalili. "However, existing hardware cannot sustain the rapid growth of data-centric computing. Our technology potentially could solve this challenge."
But before this technology can make the jump from the lab to a computer near you, Khalili says the team next needs to focus on scaling down the technology even further as well as improving its energy efficiency.
"We are working now to further downscale these devices and to improve methods to read out their magnetic state," Khalili said. "We also are looking at even more energy-efficient ways to write data into AFM materials, such as replacing the electric current with an electric voltage, a challenging task that could further increase the energy efficiency by another order of magnitude or more."
Abstract: Antiferromagnets are magnetically ordered materials without a macroscopic magnetization. As a result, they could be of use in the development of memory devices because data cannot be erased by external magnetic fields. However, this also makes it difficult to electrically control their magnetic order (Néel vector). Here, we show that pillars of antiferromagnetic PtMn, which are grown on a heavy-metal layer and have diameters down to 800 nm, can be reversibly switched between different magnetic states by electric currents. The devices are based on materials that are typically used in the magnetic memory industry, and we observe switching down to a current density of ~2 MA cm−2 . Furthermore, by varying the amplitude of the writing current, multilevel memory characteristics can be achieved. Micromagnetic simulations suggest that the different magnetic states may consist of domains separated by domain walls with vortex and anti-vortex textures that move in response to current, modifying the average Néel vector.