This AI sensor will monitor patients' hearts 24/7
With better monitoring and early intervention, this device can improve patients' quality of life after heart failure.
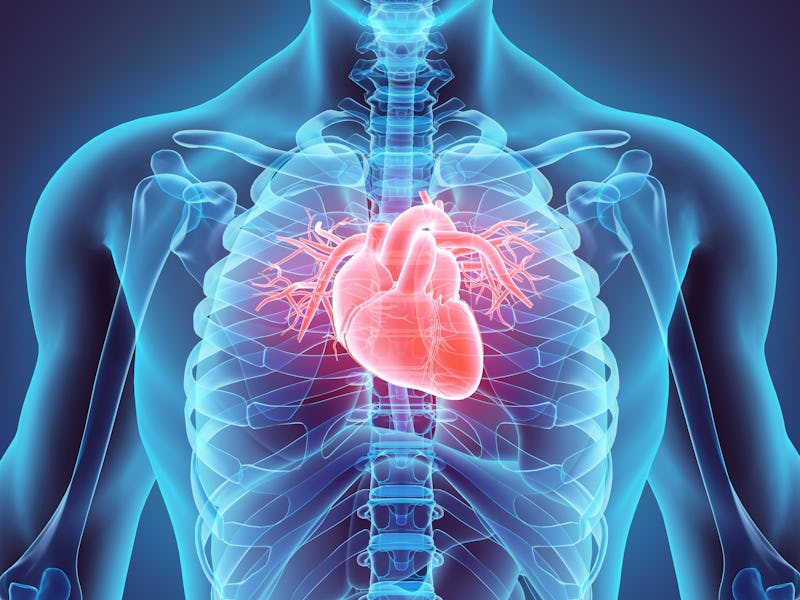
Heart failure is the top hospital discharge diagnosis in the U.S. and affects more than 23 million people worldwide. While heart failure itself isn't always deadly, its level of rehospitalization is high and can ultimately lead to a higher risk of mortality down the line. Implanted cardiac sensors, like pacemakers, have shown promise in helping patients maintain their health and independence after initial hospitalization, but the effect of less invasive cardiac monitoring technology has yet to be proven.
In a new study published Tuesday in the journal Circulation: Heart Failure researchers have demonstrated how an easy-to-use, wearable sensor driven by individualized machine learning analytics could help patients not only predict rehospitalization before it happens but also help keep patients out of the hospital altogether.
"If we can decrease this readmission rate through monitoring and early intervention, that's a big advance."
The study was conducted between August 2015 and December 2016 at hospitals in Utah, California, Texas, and Florida on a group of predominately white men in their late 60s. The group of patients, who had all been recently discharged from the hospital following a cardiac event, were given adhesive smart sensors to wear on their chests 24-hours a day for a minimum of 30 days. Using a continuous ECG the sensor was then able to collect data about the participants' skin impedance, skin temperature, and information on activity and posture to learn more about their heart rate, heart rhythm, breathing, and activity rate. The A.I. used this information to establish what each participants' healthy baseline looked like and then, as the study progressed, patients deviating from their estimated baseline would trigger the sensor.
The sensor, in turn, would send out signals to indicate their heart condition may be getting worse.
Matt Pipke, chief technology officer at the company behind the A.I. analytics used in this study, physIQ, tells Inverse that the customization of this algorithm gives this sensor a step up compared to its predecessors.
"The personalization really differentiates from past approaches which have tried to apply a threshold or heuristic rule to the population wholesale. By using a personalized model of the behavior of the patient’s vital signs in tandem, abnormalities in those vital signs can be teased out from the normal variation in vital signs that everyone experiences engaging in activities of daily living. A primary advantage is that detection may occur earlier so that there is longer lead time for the clinician to investigate patient status and intervene before a patient ends up requiring rehospitalization."
Lead author and co-chief of the advanced heart failure program at the University of Utah Health, Josef Stehlik, said in a statement that he hopes this technology can help improve monitoring and early intervention for patients with heart failure.
"There's a high risk for readmission in the 90 days after initial discharge," Stehlik says. "If we can decrease this readmission rate through monitoring and early intervention, that's a big advance. We're hoping even in patients who might be readmitted that their stays are shorter, and the overall quality of their lives will be better with the help of this technology."
This mini cardiac sensor is designed to be worn on the patient's chest 24/7 and non-invasively collect important heart data.
As this was an observational study designed to judge how accurate a sensor like this might be at evaluating rehospitalization cues, the authors of the paper did not intervene when such signals were created. They observed in the participant group that such a prediction occurred roughly 10-days before the participant was readmitted to the hospital with 80 percent accuracy. However, the device failed to make predictions prior to the death of several patients who passed away during the course of the study due to cardiac-related events.
While avoid rehospitalization may not be the right choice for every patient, Stehlik tells Inverse that he hopes it can help patients and clinicians make better-informed choices about their health.
"The prognostic algorithm detects heart failure worsening," says Stehlik. "How to use this information for treatment decisions will be in the hands of the clinicians. In some patients, outpatient treatment may not be enough and they may need to be admitted to the hospital. We believe the timely prognostic information may result in shorter admissions in those patients who do end up being admitted."
While this study was still largely a proof-of-concept, the authors write that the sensors' success in their study demonstrates a need for future testing of this approach. Going forward, the authors say they hope to have larger-scale clinical trials of these sensors and to expand their participant base to include more genders, ages, and races.
"While we do not have a reason to believe that the prognostic algorithm should be significantly affected by age," says Stehlik, "future studies should focus on enrolling more women and younger patients with heart failure."
BACKGROUND: Implantable cardiac sensors have shown promise in reducing rehospitalization for heart failure (HF), but the efficacy of noninvasive approaches has not been determined. The objective of this study was to determine the accuracy of noninvasive remote monitoring in predicting HF rehospitalization.
METHODS: The LINK-HF study (Multisensor Non-invasive Remote Monitoring for Prediction of Heart Failure Exacerbation) examined the performance of a personalized analytical platform using continuous data streams to predict rehospitalization after HF admission. Study subjects were monitored for up to 3 months using a disposable multisensor patch placed on the chest that recorded physiological data. Data were uploaded continuously via smartphone to a cloud analytics platform. Machine learning was used to design a prognostic algorithm to detect HF exacerbation. Clinical events were formally adjudicated.
RESULTS: One hundred subjects aged 68.4±10.2 years (98% male) were enrolled. After discharge, the analytical platform derived a personalized baseline model of expected physiological values. Differences between baseline model estimated vital signs and actual monitored values were used to trigger a clinical alert. There were 35 unplanned nontrauma hospitalization events, including 24 worsening HF events. The platform was able to detect precursors of hospitalization for HF exacerbation with 76% to 88% sensitivity and 85% specificity. Median time between initial alert and readmission was 6.5 (4.2–13.7) days.
CONCLUSIONS: Multivariate physiological telemetry from a wearable sensor can provide accurate early detection of impending rehospitalization with a predictive accuracy comparable to implanted devices. The clinical efficacy and generalizability of this low-cost noninvasive approach to rehospitalization mitigation should be further tested.
This article has been updated to include comments from researchers involved with this study.