Robots don’t deserve names
They don’t get “birthdays,” either.
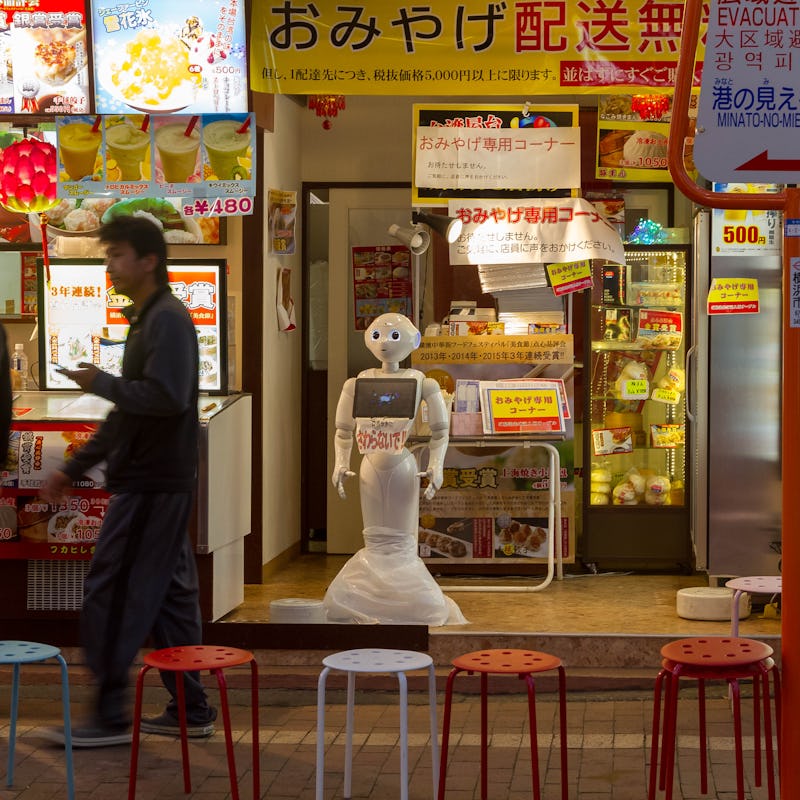
Maybe it all started with a Beanie Baby.
In 1997, McDonald’s started offering them Happy Meals. Gazing into the cream-colored bear’s glassy, unseeing eyes, you would imagine an entire life for this promotional Beanie Baby whose innards were comprised of polyester fiberfill and small plastic pellets. It had friends, passions, and a soul.
The urge to anthropomorphize non-human — and often, nonliving — things is a classic human instinct that extends far beyond childhood make-believe.
But when we project ourselves onto the technology we create, David Watson warns Inverse it could be a slippery slope. (Watson is a postdoctoral research fellow in the University College London studying machine learning.)
From humanoid service robots, and voice assistants like Siri, to the artificial intelligence powering self-driving cars, we increasingly imagine this technology in human terms.
And while telling a child or your parents that your new Tesla can “see” obstacles on the road may seem like a straightforward explanation, Cindy Grimm tells Inverse that opting for simplicity instead of precision could be more dangerous than it seems. (Grimm’s a mechanical, industrial, and manufacturing engineering professor at Oregon State University, whose work includes robot policy and ethics.)
What is anthropomorphic A.I.?
To anthropomorphize something means to project human traits or abilities onto an entity that doesn’t traditionally have them. For example, the human-animal hybrids in Bojack Horseman are anthropomorphic, and so is giving your Roomba a cute name or googly eyes.
Watson explains that anthropomorphizing is part of a human instinct to extend empathy to objects or animals we interact with daily in its simplest form. Even though your smart speaker won’t know if you raise your voice with it, you may still be polite in your requests that it set the living room lights to 50 percent.
“People dress up their Roombas.”
In addition to flexing our empathy muscles, Grimm also says that anthropomorphizing technology and A.I. is also a shortcut for helping us understand it better by applying a model that we do understand — humans — to an unknown.
“People dress up their Roombas and treat them like pets,” says Grimm. “I think that's just part of this idea called mental models, where you want to have some model of how the thing you're interacting with works ... and we tend toward building a mental model that is some version of our mental model of ourselves, which is human.”
But this is not just a human problem, says Watson; it’s also baked into the very algorithms themselves that drive much of machine intelligence today. For example, the neural networks that serve as “artificial brains” for many applications today.
“Neural networks are probably the most explicitly biomimetic of any machine learning approach,” explains Watson. “And it lends itself a lot more to overlaps with psychological research, especially with neurocognitive work.”
“I think part of what's really interesting about neural networks to people both within and beyond the machine learning community is that purported connection to human brains,” continues Watson. “[This] correspondence between biological artificial neural networks is very intriguing people, right? So they want to want to run with that.”
Grimm suggests that this kind of fascination is also being used to researchers' advantage when submitting research grants.
The downsides of anthropomorphic A.I. — On its face, anthropomorphizing may seem like a good thing. After all, what’s wrong with improving understanding and promoting empathy?
“Neural networks are not very efficient at all.”
A downside of this kind of language when it comes to A.I. is that it gives human users an incorrect understanding of what technology can and cannot do. This could be inconvenient if you expect Siri to understand your take-out preferences, or even potentially deadly when it comes to our self-driving cars, says Grimm.
“Let’s say you get into a car, and you're told that the car has automated cruise control,” says Grimm. “And so the person gets up and walks into the back of their RV because it's automated cruise control.
“But if you had said the car could maintain a constant speed with a car in front of it, but it isn't going to handle very well when somebody cuts in front of you, or the lanes disappear, that's a different conversation to be having.”
“When you have that mismatch between what the robot is actually capable of doing and when you use these human terms, you instantly jump to this human level capability,” Grimm adds.
Watson says that neural networks are also far from the best option for artificial intelligence, despite the popularity (among academics and the public) the technique has gained over the years.
“Neural networks are not very efficient at all,” Watson says. “Because the learning procedure itself has to be sequential.”
The ethics of humanlike A.I.
Another problem with anthropomorphic A.I., says Watson, is that it blurs the lines of responsibility when making crucial human decisions, like whether or not somebody is guilty of a crime.
Machine learning robots aren’t just helping us drive or clean our house but they’re helping us make decisions about the future of people’s lives.
Just because an A.I. may be good at finding patterns or flaws in a witness’s testimony doesn’t mean this technology has moral agency, argues Watson.
“It's a total abdication of responsibility on the part of the humans who inevitably were involved in the design and deployment of that algorithm,” says Watson. “At juncture points in between that algorithm not existing and that algorithm being involved in some criminal sentencing case, there were human decisions that sped that along — at so many points.”
Grimm says that expectations of privacy are also an issue when it comes to A.I. technology that uses anthropomorphic language to obscure how it truly works.
For example, a Roomba doesn’t simply “see” your house the way a human does, explains Grimm. Instead, it takes grainy photos and videos to upload to the cloud, often without customers realizing it.
The alternative to naming your Roomba — While we may be truly entangled in this web of anthropomorphic tech today, that doesn’t have to mean it's a permanent reality.
Watson explains that plenty of other viable existing options could be used in place of this supervised learning method when it comes to machine learning frameworks. Methods such as lasso, bagging, and boosting — which are examples of linear and ensemble models, he says — could be both more computationally effective than traditional neural networks as well as less reliant on mimicking biological structure.
As for how we describe our bots and existing A.I. tech, Grimm says we should bite the bullet and go cold turkey off the anthropomorphic language. We’ll be thankful for it in the long run. Roombas are amazing. They steadily clean your floors while you go about your day or even while you sleep. But maybe you shouldn’t name it.
A screenshot from the iRobot app shows where you can name your robot, and its “birthday.”
“We would just do away with it,” says Grimm. “It’s a shortcut, and like many shortcuts, it is not very helpful.”