You could soon be texting with just your mind, thanks to new research
This innovation would help improve brain-machine interfaces with those with speech disorders.
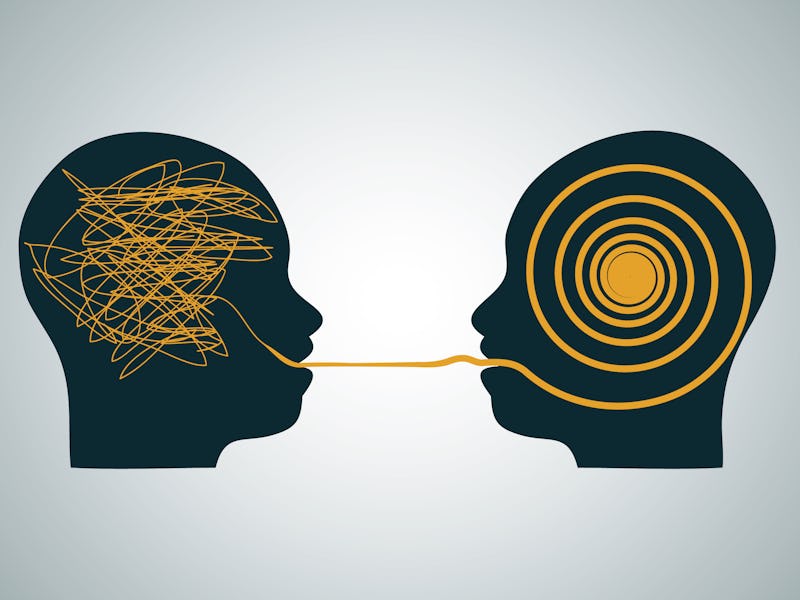
Reading minds sounds like the stuff of fiction—but to speech therapists, it has a powerful therapeutic potential. And now a new machine learning model has brought this technology closer to reality than ever.
Using two different neural networks and intracranial electrodes, computer scientists and neuroscientists from UC San Francisco were able to translate neural activity into text with as low as three percent error. The authors write that translations like these could be used to further develop brain-machine interfaces for patients with speech disorders.
In the study, published Monday in the journal Nature Neuroscience, the authors write that their approach is similar to something many have everyday experience with: language translation apps.
"To achieve higher accuracies, we exploit the conceptual similarity of the task of decoding speech from neural activity to the task of machine translation; that is, the algorithmic translation of text from one language to another," write the authors. "Conceptually, the goal in both cases is to build a map between two different representations of the same underlying unit of analysis."
The authors write that focusing on translating neural signals into words instead of syntactic chunks (as has been done in previous research) allowed them to achieve higher accuracies without needing to expand the vocabulary used in their study.
However, the study's lead author and associate neurological surgery researcher at UC San Francisco, Joseph Makin, tells Inverse that while their model may be more accurate than previous approaches, it's still far from foolproof.
"This is much better than previous results (word error rates around 60%), but it's important to emphasize that the decoder does not generalize very well, at this point, to novel sentences," says Makin.
To test this method, the researchers collected data from four participants as they read aloud a list of 30 to 50 sentences. Because these four participants happened to be receiving seizure monitoring and had intracranial electrodes implanted in their brains already, the researchers were able to collect data on both their neural activity as well as auditory data of the spoken sentences.
Neural signals collected by intracranial electrodes are passed through two neural networks that make a prediction about what words are being spoken.
To translate, the neural activity collected from participants was fed into a first neural network that combed through the data to look for signals that might be connected to speech, such as commands to move a participants' mouth or repeated signals that could represent parts of speech like vowels or consonants. This collection of speech-related signals was then passed to a second neural network that predicted what word the signals might be connected with.
When compared to the actual sentences spoken by participants the researchers found their model had error rates as low as three percent, nearly equivalent to the accuracy of professional-level speech transcription algorithms.
But, not always. Some particularly amusing incorrect predictions included confusing the phrase "those musicians harmonize marvelously" for "the spinach was a famous singer" and confusing "those thieves stole thirty jewels" for "which theatre shows mother goose."
Based on the success of this model with only half an hour of data, the researchers are hopeful that a similar system implanted permanently in chronic speech disorder patients would greatly expand the vocabulary and flexibility of this model.
"In the long run, we think people who have lost speech--from ALS, a stroke, or some other traumatic brain injury--but remain cognitively intact would benefit from a speech prostheses along the lines of the set up in this study," Makin tells Inverse. "But that's at least several years into the future."
Abstract: A decade after speech was first decoded from human brain signals, accuracy and speed remain far below that of natural speech. Here we show how to decode the electrocorticogram with high accuracy and at natural-speech rates. Taking a cue from recent advances in machine translation, we train a recurrent neural network to encode each sentence-length sequence of neural activity into an abstract representation, and then to decode this representation, word by word, into an English sentence. For each participant, data consist of several spoken repeats of a set of 30–50 sentences, along with the contemporaneous signals from ~250 electrodes distributed over peri-Sylvian cortices. Average word error rates across a held-out repeat set are as low as 3%. Finally, we show how decoding with limited data can be improved with transfer learning, by training certain layers of the network under multiple participants’ data.