Why Fitbits may be the key to stopping deadly infections in their tracks
The technology may be a vital weapon in the race to prevent the next "Contagion."
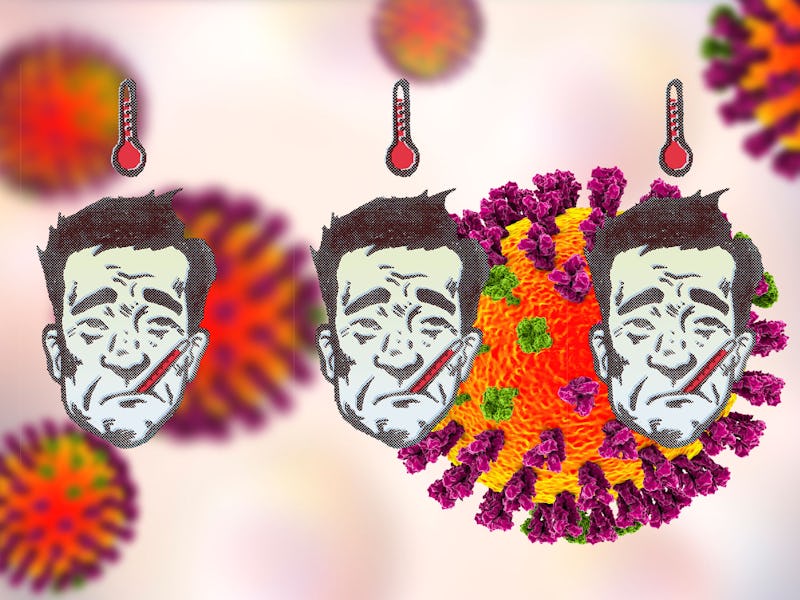
Influenza is notoriously hard to track — flu strains are both unpredictable and highly contagious, spreading like wildfire across communities. But wearable technology like Fitbits could hand epidemiologists the upper hand. New research suggests the fitness trackers may hold the key to tracing — and predicting — flu outbreaks in real time.
In a study, published Thursday in The Lancet Digital Health Journal, researchers harness FitBit users’ sleep and heart rate data to pinpoint exactly when certain states were getting hit by the flu. They were then able to predict where the outbreaks might spread more accurately and rapidly than conventional methods, the researchers say.
Traditional flu tracking has a one to three week-long lag. The delay in tracking can allow outbreaks to go “unnoticed,” the researchers say, enabling the virus to spread through new populations. By contrast, wearable tech data may offer accurate measurements instantaneously.
“In the future as these devices improve, and with access to 24/7 real-time data, it may be possible to identify rates of influenza on a daily instead of weekly basis,” Jennifer Radin, study co-author and epidemiologist at the Scripps Research Translational Institute, said in a press release.
The stakes are high: Influenza may be responsible for as many as 49 million incidences of illness every year in the United States, according to the United States Centers for Disease Control and Prevention (CDC).
Data dump
The study included 200,000 FitBit users who wore the fitness tracker between the period March 1, 2016 until March 1, 2018. From this sample, the team focused on 47,248 users from California, Texas, New York, Illinois and Pennsylvania who wore the device consistently during the study period.
The average user was 43 years old, and 60 percent were female — other demographic information was stripped for the purposes of the study. Fitbit users are notified that their de-identified data could potentially be used for research in the Fitbit Privacy Policy.
Over the course of the study, the 47,248 users yielded a data set of over 13 million daily measurements, including their sleep times, heart rate, and Fitbit use.
The Fitbit has long offered native sleep tracking, but its accuracy is disputed.
The idea that Fitbits can help track the flu hinges on two of the body’s physiological responses to infection: spiked heart rate and longer sleep. When someone is fighting off the flu, their resting heart rate goes up and they are likely to sleep for longer than they might usually.
By comparing moments when users’ sleep and heart rate deviated from the norm with the CDC’s estimates of “influenza-like-incidence”, the researchers were able to estimate how many people had the flu in each state during any given week.
To check their numbers, the researchers compared their data to confirmed flu rates established by the CDC at the end of the flu season.
Influenza incidence predictions in all five states were more accurate when Fitbit data was incorporated into the models than when it was left out. Overall, Fitbit data improved accuracy between 6.3 and 32 percent. At the same time, all five states saw an improvement in “real-time surveillance,” according to the study.
But just how much faster and better these predictions would be as compared to traditional methods on a country-wide — or international — scale isn’t clear.
Limits to the data
The study has some limitations: Fitbit wearers are likely to be wealthier and healthier than the general population, with greater access to flu vaccines and fewer conditions compounding their risk of illness.
And because the study used anonymized individuals’ data, it’s impossible to know if the heightened resting heart rate and longer sleep periods recorded were in response to flu or in response to a host of other viral and bacterial infections.
The CDC estimates that flu is responsible for up to 49 million illnesses each year in the US.
At the same time, sleep-tracking devices don’t always give accurate readings, a technological failure that could have skewed the results.
Resting heart rate and sleeping patterns also change with the seasons — during winter, people are likely to sleep longer and be dehydrated, which can elevate heart rate. Winter also happens to be peak flu season.
More research that factors in demographic differences and takes other factors like seasonal fluctuations into account is needed before wearable tech can be used to reliably predict flu outbreaks in a meaningful way.
In the past, researchers have tried to use other crowd-sourcing methods as prediction tools — including mining data from Google and Twitter search trends. But the results have been mixed and often overestimate influenza rates. It is difficult to break down who is actually infected with the flu and looking for information online, versus those who have family or friends with the flu and are searching the web.
But the research does suggest that wearables may be a powerful tool to add to the arsenal of public health officials. This will depend on whether researchers can refine some of these wearable trackers’ technological weaknesses and if companies determine ways to protect the privacy of users. Looking to the future, if we can get wearables to work for the flu, then they may be useful in tracking other kinds of disease outbreaks, too — especially in places where traditional health monitoring is difficult, such as war zones and rural regions.
Abstract:
Background Acute infections can cause an individual to have an elevated resting heart rate (RHR) and change their routine daily activities due to the physiological response to the inflammatory insult. Consequently, we aimed to evaluate if population trends of seasonal respiratory infections, such as influenza, could be identified through wearable sensors that collect RHR and sleep data.
>Methods We obtained de-identified sensor data from 200 000 individuals who used a Fitbit wearable device from March 1, 2016, to March 1, 2018, in the USA. We included users who wore a Fitbit for at least 60 days and used the same wearable throughout the entire period, and focused on the top five states with the most Fitbit users in the dataset: California, Texas, New York, Illinois, and Pennsylvania. Inclusion criteria included having a self-reported birth year between 1930 and 2004, height greater than 1 m, and weight greater than 20 kg. We excluded daily measurements with missing RHR, missing wear time, and wear time less than 1000 min per day. We compared sensor data with weekly estimates of influenza-like illness (ILI) rates at the state level, as reported by the US Centers for Disease Control and Prevention (CDC), by identifying weeks in which Fitbit users displayed elevated RHRs and increased sleep levels. For each state, we modelled ILI case counts with a negative binomial model that included 3-week lagged CDC ILI rate data (null model) and the proportion of weekly Fitbit users with elevated RHR and increased sleep duration above a specified threshold (full model). We also evaluated weekly change in ILI rate by linear regression using change in proportion of elevated Fitbit data. Pearson correlation was used to compare predicted versus CDC reported ILI rates.
>Findings We identified 47 249 users in the top five states who wore a Fitbit consistently during the study period, including more than 13·3 million total RHR and sleep measures. We found the Fitbit data significantly improved ILI predictions in all five states, with an average increase in Pearson correlation of 0·12 (SD 0·07) over baseline models, corresponding to an improvement of 6·3–32·9%. Correlations of the final models with the CDC ILI rates ranged from 0·84 to 0·97. Week-to-week changes in the proportion of Fitbit users with abnormal data were associated with week-to-week changes in ILI rates in most cases.
>Interpretation Activity and physiological trackers are increasingly used in the USA and globally to monitor individual health. By accessing these data, it could be possible to improve real-time and geographically refined influenza surveillance. This information could be vital to enact timely outbreak response measures to prevent further transmission of influenza cases during outbreaks.