Scientists are using A.I. to unlock humanity's most mysterious mutations
It's already catching disease-causing mutations, and it's just getting started.
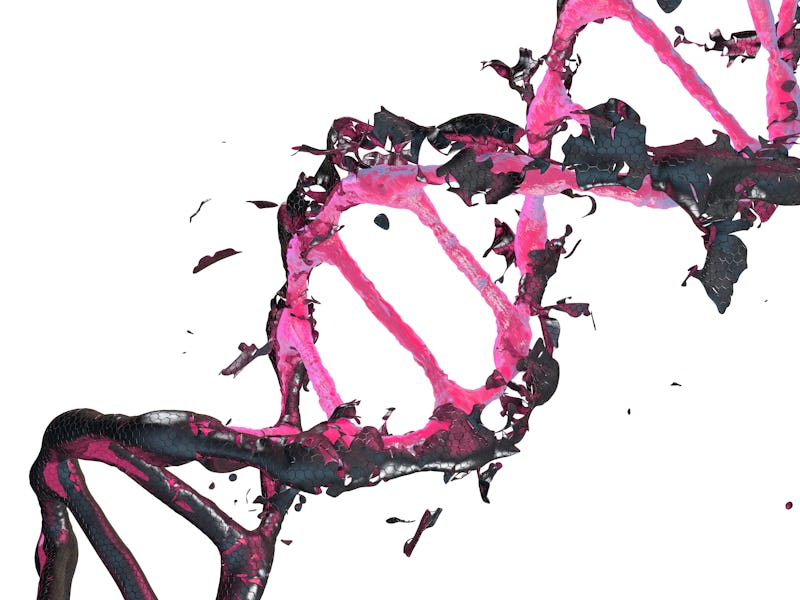
Genetic mutations take place deep inside our DNA and can be challenging to identify, let alone treat. Scientists hope that a new deep learning approach will help doctors better combat these disease-causing mutations.
Thanks to their data-crunching abilities, deep learning and A.I. have become increasingly important medical tools in recent years. These models are able to digest and make use of reams of medical data created by the human body by learning patterns from a test data-set and applying those rules to new, incoming data. Far from replacing a physician, these medical machines simply help physicians make connections quicker and more accurately.
While previous deep learning approaches have found success in predicting harmful mutations in the human genome, this new approach is the first to target metal-binding sites of proteins.
The study was published this December in the journal Nature Machine Intelligence and used something called a multichannel convolutional neural network (MCNN) to better understand what kinds of mutations affect disease development. Because metal ions play key structural and physiological roles in the human body, the team focused specifically on the regulation of different metallic nutrients in proteins, something called metalloproteins.
The study’s lead author, Hongzhe Sun, a professor and chemistry chair at the University of Hong Kong, said in a statement that this approach can make use of data the team has already collected.
“Machine learning and AI play important roles in the current biological and chemical science,” said Sun. “In my group we worked on metals in biology and medicine using integrative omics approach including metallomics and metalloproteomics, and we already produced a large amount of valuable data using in vivo/vitro experiments. We now develop an artificial intelligence approach based on deep learning to turn these raw data to valuable knowledge, leading to uncover secrets behind the diseases and to fight with them. I believe this novel deep learning approach can be used in other projects, which is undergoing in our laboratory.”
The MCNN's work flow when crunching data.
But, before bringing in the A.I., the team first had to analyze data collected from these metalloproteins. They found that mutations in different metal ions, usually caused by a change in size or hydrophilic-ness, affected the development of different diseases. For example, zinc-binding site mutations appeared to play a major role in breast, liver, kidney, immune system and prostate diseases while mutations in calcium- and magnesium-binding sites were associated with muscular and immune system diseases. Due to data availability, the research focused on these three metal types.
From there the researchers broke their data into 80 percent training data —for the MCNN to learn from — and 20 percent testing data — to determine how well the MCNN could apply its new knowledge to novel situations. In order to gain useful knowledge from the data sets, the team extracted both spatial and sequential features from the data and fed that to the MCNN.
The research team (from left) Dr Haibo Wang, Dr Mohamad Koohi-Moghadam, Professor Hongzhe Sun and Dr Hongyan Li at the Department of Chemistry, The University of Hong Kong
Using this data the MCNN was able to identify two disease-causing mutations that a previous similar study, PolyPhen-2, had only marked as benign. These mutations were connected to a variety of cancers as well as a rare genetic disorder called Johanson–Blizzard syndrome. Apart from these two novel discoveries, the team also found that the MCNN was able to correctly identify disease-causing mutations 82 percent of the time.
In addition to being a useful tool to help researchers make sense of genetic data and to better tackle disease-causing mutations, the research team also hopes that their approach could be used to develop new drugs as well by predicting the binding affinity of small molecules and proteins.
Abstract:
Metalloproteins play important roles in many biological processes. Mutations at the metal-binding sites may functionally disrupt metalloproteins, initiating severe diseases; however, there seemed to be no effective approach to predict such mutations until now. Here we develop a deep learning approach to successfully predict disease-associated mutations that occur at the metal-binding sites of metalloproteins. We generate energy-based affinity grid maps and physiochemical features of the metal-binding pockets (obtained from different databases as spatial and sequential features) and subsequently implement these features into a multichannel convolutional neural network. After training the model, the multichannel convolutional neural network can successfully predict disease-associated mutations that occur at the first and second coordination spheres of zinc-binding sites with an area under the curve of 0.90 and an accuracy of 0.82. Our approach stands for the first deep learning approach for the prediction of disease-associated metal-relevant site mutations in metalloproteins, providing a new platform to tackle human diseases.