Self-driving cars could increase capacity 138% with this one weird trick
Smart cars need help. Say hello to the smart intersection.
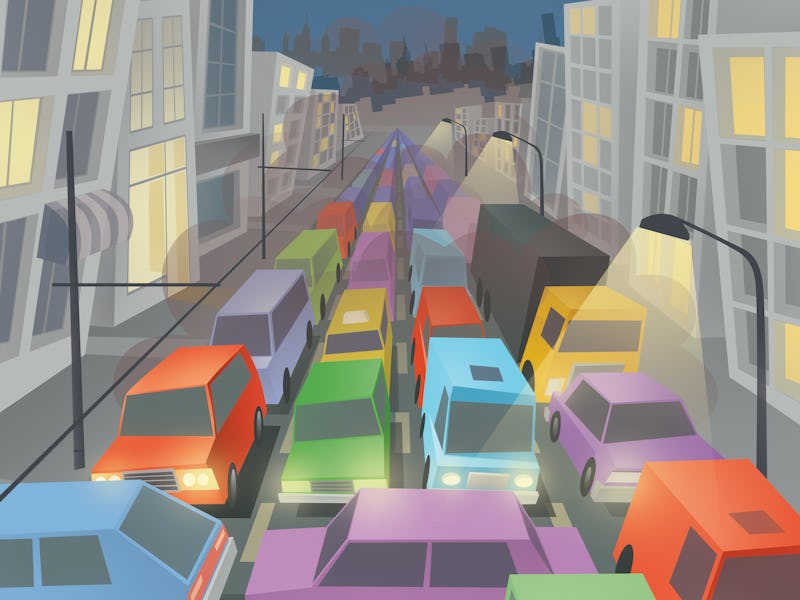
Smart intersections could co-operate with self-driving cars to make traffic flow smoother than ever, according to research published this week.
A team at Cornell University developed a model to allow platoons of vehicles to move through intersections with no wait times. The tests showed that when cars and infrastructure can work together, the autonomous vehicle can enable far smoother traffic flow. The findings were published December 15 in Transportation Research Part B.
“Here, the rise in the capacity is 138 percent,” Oliver Gao, professor of civil and environmental engineering and a senior author of the paper, tells Inverse. “We modeled the system at its extreme point of best achievable results.”
The model was based around assuming that only autonomous cars were on the road, allowing a very small gap size to ensure the system could cope with unexpected errors. That means the 138 percent figure is a best-case scenario. The figures will vary when non-autonomous cars and other vehicles like bikes enter the mix.
“Yes, smartphone-enabled pedestrians and bikes can be considered in the mode while AVs are equipped with advanced visual analyzer systems,” Gao says.
As a potential vision for the future of transport, the concept could enable vehicles to use the same space faster than ever.
Google's prototype self-driving car.
Self-driving cars of the future: how it works
The autonomous car promises to take the human driver out of the equation and let a machine do the work. This could potentially reduce the gaps in traffic. In a 2016 video, YouTuber “CGPGrey” explained how drivers braking creates traffic ripples that causes long-lasting slowdowns, the sort of problem that would be solved by machines running the show:
Researchers have produced models for optimizing intersections, but this one focuses specifically on how the autonomous car could change the situation. The smart intersection in Gao’s scenario works with the cars to ensure the infrastructure helps cars move through at speed.
“Instead of having a fixed green or red light at the intersection, these cycles can be adjusted dynamically,” Gao said in a statement. “And this control can be adjusted to allow for platoons of cars to pass.”
The simulation looked at two variables. The first is platoon size, or how many autonomous cars move as one in a tightly-formed pack. The second is marginal gap length between the departure and arrival through the intersection, creating a gap that allows for issues with synchronization. Balancing these two variables led the team to the 138 percent figure.
It sounds like a good solution to the gridlock of major cities, but other research shows it might not be so simple. A February 2019 analysis published in Transport Policy noted that autonomous cars could freely roam the streets to avoid parking fines. Even if a small fraction of people took advantage of this capability, it could be enough to cause chaos. In the simulation, adding 2,000 cars to downtown San Francisco ground traffic to an excruciating two miles per hour.
The solution could be a complete ban on cars in downtown areas. Amos Haggiag, CEO of the transportation startup Optibus, told Inverse in February 2018 that, faced with a slew of inefficiently-sized boxes driving around, cities will begin to “massively and probably aggressively favor mass transportation over taxis and private vehicles.”
With autonomous vehicles set to hit the road in the 2020s — depending on whether automakers overpromise and under-deliver again — cities have some time left to work out the answer to questions like these.
Abstract:
Recent advances in artificial intelligence and wireless communication technologies have created great potential to reduce congestion in urban networks. In this research, we develop a stochastic analytical model for optimal control of communicant autonomous vehicles (CAVs) at smart intersections. We present the automated network fundamental diagram (ANFD) as a macro-level modeling tool for urban networks with smart intersections. In the proposed cooperative control strategy, we make use of the headway between the CAV platoons in each direction for consecutive passage of the platoons in the crossing direction through non-signalized intersections with no delay. For this to happen, the arrival and departure of platoons in crossing directions need to be synchronized. To improve system robustness (synchronization success probability), we allow a marginal gap between arrival and departure of the consecutive platoons in crossing directions to make up for operational error in the synchronization process. We then develop a stochastic traffic model for the smart intersections. Our results show that the effects of increasing the platoon size and the marginal gap length on the network capacity are not always positive. In fact, the capacity can be maximized by optimizing these cooperative control variables. We analytically solve the traffic optimization problem for the platoon size and marginal gap length and derive a closed-form solution for a normal distribution of the operational error. The performance of the network with smart intersections is presented by a stochastic ANFD, derived analytically and verified numerically using the results of a simulation model. The simulation results show that optimizing the control variables increases the capacity by 138% when the error standard deviation is 0.1 s.
Update 12/20 11 a.m. Eastern time: An earlier version of this article stated that the system would reduce congestion by 138 percent. It has since been amended.