A.I. could help predict if historic bridge will collapse
They're beautiful. They're working long past their time. They could be deadly.
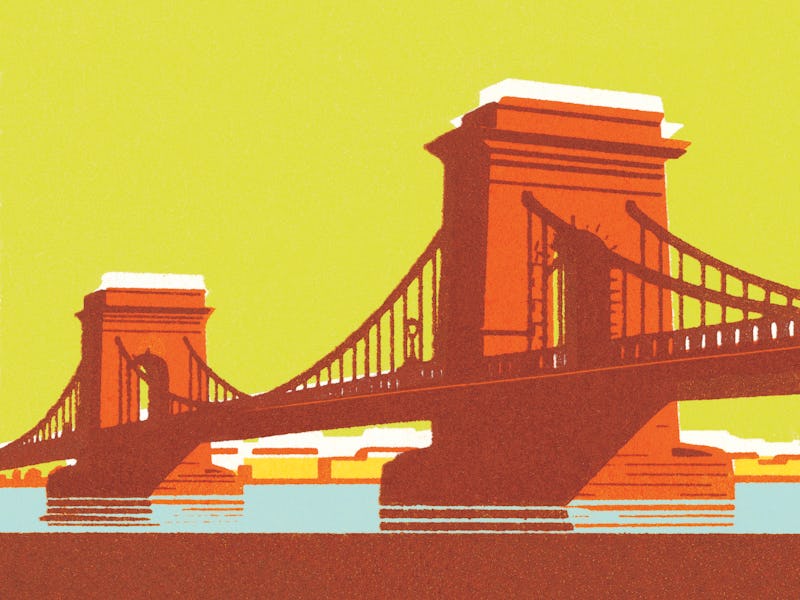
The bridges of the future could use artificial intelligence to stay up stronger for longer.
A team from the University of Surrey and King’s College London have developed an A.I. system dubbed SHMnet that can understand the subtle changes in structures over time, identifying when a bridge is in danger. The findings were published Sunday in the journal Structural Health Monitoring.
“The performance of our neural network suggests that SHMnet could be incredibly useful to structural engineers, governments and other organizations tasked with monitoring the integrity of bridges, towers, dams and other metal structures,” Ying Wang, assistant professor at the University of Sydney, said in a statement.
If successful, it could help pre-empt major issues in some of the world’s biggest structures. These impressive old structures have withstood the test of time, but some require maintenance to continue supporting daily traffic. As the 2019 fire at the Notre Dame Cathedral showed, older structures can pose unique risks. The historical structures could receive a helping hand from a decidedly cutting-edge source.
London's Tower Bridge: about to fall down?
How A.I. can supercharge the bridge
Bridge maintenance is a key area of research, as some of them have stood for centuries but still maintain regular traffic. The United Kingdom’s rail network uses a number of bridges from the Victorian era, for example. Some bridges far outlast their originally-designed lifespan, providing good quality service but also raising questions about maintenance.
These issues came to the forefront in August 2018, when the Morangi bridge in the Italian town of Genoa collapsed. Marios Chryssanthopoulos, professor of structural systems at the University of Surrey, noted in his story for The Conversation that “considering the number of bridges built in Europe during the expansion of the motorway networks from the late-1950s onwards, we should expect, and be prepared for, many to exceed their planned lifespan in coming decades.”
Tools like SHMnet could shed some light on the state of specific bridges. The system used an Alex-Net neural network to look at the connection bolts on a steel frame as part of 10 damage scenarios. When trained on four datasets, the network had an impressive 100 percent identification rate.
“While there is more to do, such as testing SHMnet under different vibration conditions and obtaining more training data, the real test is for this system to be used in the field where a reliable, accurate and affordable way of monitoring infrastructure is sorely needed,” Wang said.
Researchers have been exploring how to use A.I. to beat humans at classic video games and perhaps even remove ads from everyday podcasts. But with the Surrey team’s creation, A.I. could also playing a role in ensuring the structural integrity of critical infrastructure.
>Abstract
Deep learning algorithms are transforming a variety of research areas with accuracy levels that the traditional methods cannot compete with. Recently, increasingly more research efforts have been put into the structural health monitoring domain. In this work, we propose a new deep convolutional neural network, namely SHMnet, for a challenging structural condition identification case, that is, steel frame with bolted connection damage. We perform systematic studies on the optimisation of network architecture and the preparation of the training data. In the laboratory, repeated impact hammer tests are conducted on a steel frame with different bolted connection damage scenarios, as small as one bolt loosened. The time-domain monitoring data from a single accelerometer are used for training. We conduct parametric studies on different layer numbers, different sensor locations, the quantity of the training datasets and noise levels. The results show that the proposed SHMnet is effective and reliable with at least four independent training datasets and by avoiding vibration node points as sensor locations. Under up to 60% additive Gaussian noise, the average identification accuracy is over 98%. In comparison, the traditional methods based on the identified modal parameters inevitably fail due to the unnoticeable changes of identified natural frequencies and mode shapes. The results provide confidence in using the developed method as an effective structural condition identification framework. It has the potential to transform the structural health monitoring practice. The code and relevant information can be found at https://github.com/capepoint/SHMnet.