Friends or FitBit? 4 Areas Where Both Play a Critical Role in Your Health
Close friends are likely to have similar fitness tracker metrics, a new study has found.
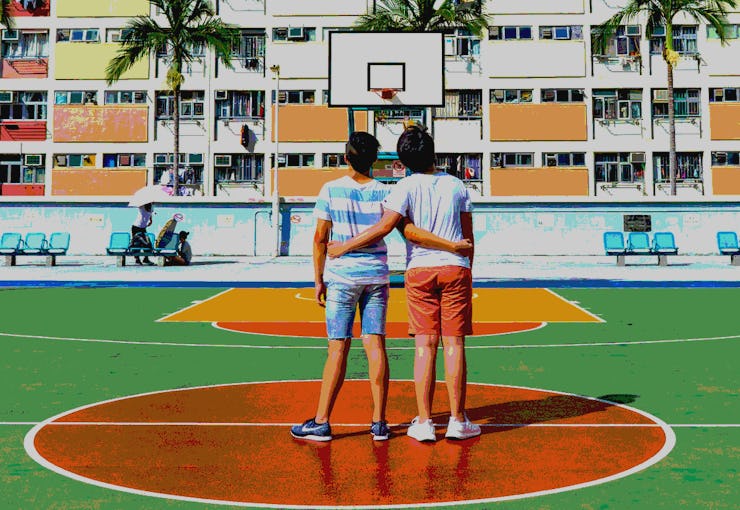
Researchers have long attempted to boil healthiness down to a single, memorable metric. There’s always been the number of pounds. Then came the 2,000-calorie daily diet. Today, wearables have emerged as the latest focus for data. There are step counters and stress trackers. But new research points to a surprisingly good, and very old, health indicator that you won’t find on a weigh scale, a nutrition label, or your wrist. It’s the structure of your friend group.
In a paper published June 6 in PLOS ONE, a team of scientists showed that data describing someone’s social network can add valuable insight into their physical and mental health.
Nitesh Chawla, Ph.D., a computer engineering professor at Notre Dame, showed that social network structures have strong ties to health metrics like steps, heart rate, or activities during the day. Chawla’s data illuminated a pattern championed by online fitness communities and workout groups alike: If your friends are active, there is a pretty good chance you will be too.
“Imagine the context of having a gym buddy or group,” Chawla tells Inverse. “There is that support, that understanding, that motivation, and that perception of self and others that helps us develop that social network support.”
Chawla’s findings go beyond the power of a workout buddy. Social network information can predict student happiness, positivity, stress levels, and self-reported health more precisely than just fitness tracker data.
Social network data could predict levels of self-reported health in college students, and was correlated with health behavior data, like steps or activity during the day.
Chawa’s study is based on health and friendship data from 698 Notre Dame freshmen. As part of the study, the freshmen each wore a FitBit Charge HR, which tracked heart rate, daily steps, and activity states for each student. Next, Chawa analyzed texts and phone calls that were captured through an app on their phones to collect information about each student’s social network.
Chawla analyzed those texts and calls according to variables that were intended to show how involved each student was in their own social world. For instance, he analyzed how connected someone was to their social network, or the level of intimacy among the group as whole. From there, he was established a statistically significant relationship between social network structures and health behaviors.
Essentially, close friends are likely to have similar fitness tracker metrics.
The “Network Effect”
If your friends share the same qualities to you — like fitness tracker metrics — it illustrates a hypothesis called the “network effect,” an idea that “similarities in lifestyle and health practice, including health behavior, moods, emotions, cultural norms, etc., among individuals is also a result of influence and diffusion within their network through their ties.” The more popular application of the network effect, is through an economic lens; when more people use a service (Twitter or Uber), the more valuable the service becomes for everyone.
All of which is to say: Your behavior is really affected by the actions of your friends. Certain activities from health behaviors to moods or cultural norms can “diffuse” over social networks, the authors write.
In this case, Chawla explains that his findings suggest that activities that influence health, like movement, for instance, can diffuse throughout a friendship network, too. If social network really is so intimately tied to health behaviors, the next phase of his experiment put it to the test.
The Results
Chawla’s algorithm showed that friendship data helps predict certain levels of health and wellness in college students.
For example, when he combined combined social network analysis — those texts and phone calls — with FitBit data, he found increases in predictability of five areas:
1. Happiness
He was able to predict student happiness with 65 percent greater accuracy than with just FitBit data.
2. Attitude
The accuracy of predicting a student’s positive attitude also increased by 54 percent.
3. Stress
He was 38 percent more accurate in predicting stress levels than if he had only used a student’s FitBit data.
4. Student’s Self-Assessment of Health
Chawla was also able to predict how healthy a student thought they were with 55 percent greater accuracy.
Algorithms aside, Chawla’s findings are partially illustrative of his own experience. While summiting Mount Killamanjaro, he notes that it was both the training, and the social network of his climbing partners that go him to the top in equal measure:
“It was more about the support we received from each other during that versus the individual fitness levels. A couple of my friends struggled toward the end, but the network pushed them on. While I captured a ton of data on my fitness trackers, but the summit was a factor of my training and my social network,” he says.
With that in mind, work seems like pretty good reason to bring a friend along for a run or climb. Investing in the health of your friends, over time, may prove to be a useful investment in yourself as well.
Abstract:
Social networks influence health-related behavior, such as obesity and smoking. While researchers have studied social networks as a driver for diffusion of influences and behavior, it is less understood how the structure or topology of the network, in itself, impacts an individual’s health behavior and wellness state. In this paper, we investigate whether the structure or topology of a social network offers additional insight and predictability on an individual’s health and wellness. We develop a method called the Network-Driven health predictor (NetCARE) that leverages features representative of social network structure. Using a large longitudinal data set of students enrolled in the NetHealth study at the University of Notre Dame, we show that the NetCARE method improves the overall prediction performance over the baseline models—that use demographics and physical attributes—by 38%, 65%, 55%, and 54% for the wellness states—stress, happiness, positive attitude, and self-assessed health—considered in this paper.