2 Simple Mental Tricks Helped Liars Beat a Brain-Scanning Lie Detector Test
This setback is helping scientists make an unbeatable test.
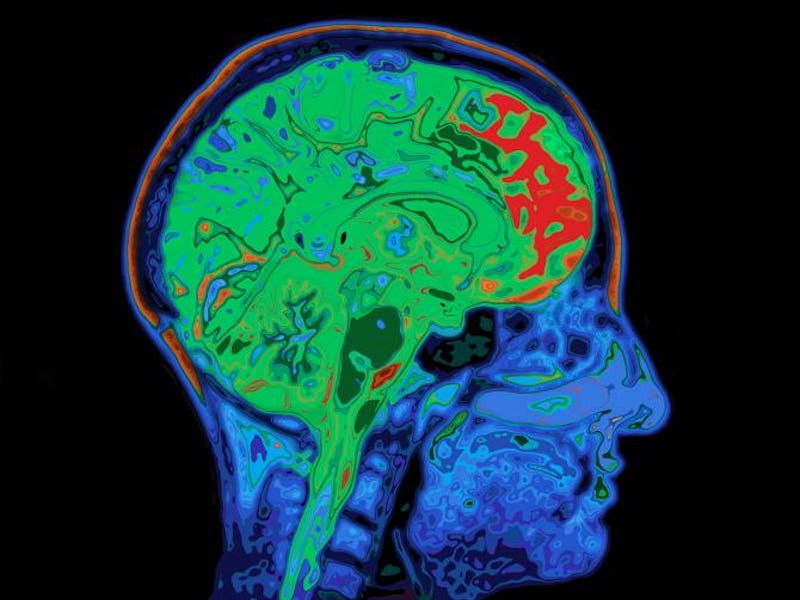
Good liars can control every physical clue of deception: sweaty palms, a shifting glance? No problem. But the best liars know how to control the signs of lying in the brain, and they do it so well that they can even fool brain scanning technology. In a dystopian future, in which bad actors try to extract state secrets from you using brain imaging, think back to this paper in Human Brain Mapping, which outlines the best way to beat the test.
The type of lie detector test used in this paper is an fMRI imaging technique. fMRI scans are currently used to map blood flow in the brain and have led to great insights into how the brain affects behavior, but it has also been controversially proposed as a lie detector technique. Famously, use of fMRI-based lie detector tests were shot down in a Tennessee court case in 2010, partially because there are questions about their accuracy in real-life situations, a concern that has been echoed in many analyses since then.
"If we ever want to try to use these methods in the courtroom, then we must know exactly what their accuracy and weaknesses are."
Just because these tests aren’t ready for primetime yet, that doesn’t mean they won’t be later, says Giorgio Ganis, Ph.D., a cognitive neuroscientist at the University of Plymouth and the lead author of this paper.
“If we ever want to try to use these methods in the courtroom, then we must know exactly what their accuracy and weaknesses are,” he tells Inverse.
How to Beat the fMRI Lie Detector Test
Ganis’ experiment was focused on 20 participants, who were told to conceal information from the experimenters. The “liars” were told a secret number ahead of time. Then, they were shown lists of numbers on a computer screen and were told to lie about whether the number shown on the screen was the one they were told ahead of time. This is sometimes called a concealed information test.
Past lie detector tests investigated physiological consequences of lying, but in the future, we may look for signals of deception in the brain.
Concealed information tests are occasionally used by the FBI as interrogation techniques. For example, instead of asking you “did you stab that man?” someone employing a concealed information test may ask about specific details and look for signs of familiarity. For instance, they may ask: was there a gun at the scene? A knife?
Ganis explains that two things happen when someone is confronted with this information. Someone guilty of the crime will recognize an item from the crime scene. Then, their brain will work extra hard to conceal that recognition. fMRI-based lie detector tests search for brain signals that indicate those processes.
“Both processes activate certain brain regions, especially in the prefrontal cortex. Innocent people will not show this response since they would not know what the crime item is, they were not there,” says Ganis. “So, we can use these brain signals to figure out if someone is familiar with the crime items, even if this person lies about it and claims no knowledge.”
But in his experiment, Ganis proposes two ways to help the brain scramble these clear patterns of familiarity.
First, researchers told volunteers to focus on the superficial, physical attributes of the number they were shown. So if you were trying to conceal that your secret number was 5, and the number 5 flashed on the screen you might focus on the smoothness of the sans-serif font or the actual color of the number on the screen.
Participants had to try to conceal their familiarity with a certain number using two techniques.
An additional technique that the liars were told to employ was to try to attach emotional connections to the irrelevant numbers. So if you’re trying to conceal the fact that the number five has a special meaning, this technique would have you attach similar importance to a random number, say seven.
Ganis’ results showed that these techniques were effective enough to beat the brain scanning lie detectors. Overall, the accuracy of the test decreased by 20 percent when the liars employed these techniques.
The Future of Brain-Based Lie Detector Tests
Importantly, the use of brain-based lie detector tests is still emerging — it’s not something we’re likely to see right away. Right now, Ganis acknowledges that accuracy is a stumbling block.
“Currently, fMRI scans for detecting concealed knowledge and lies are not reliably better than using measures such as skin conductance, like sweating,” he says. “If you want to give it a number, you could say that we are in the high 80 percent accuracy in the lab.”
Interestingly, studies like Ganis’ may actually help pave the way for these tests in the future. “Clearly, if someone can ‘beat the test’, then the test cannot be used as-is,” he notes. “Ideally, we should design tests that are resistant to countermeasures.”
In this paper, Ganis has revealed a major achilles heel that could one day build a truly reliable brain-based fMRI test. If that day arrives the people who will try to beat that test will at least have somewhere to start.
Abstract: During the last decade and a half, functional magnetic resonance imaging (fMRI) has been used to determine whether it is possible to detect concealed knowledge by examining brain activation patterns, with mixed results. Concealed information tests rely on the logic that a familiar item (probe) elicits a stronger response than unfamiliar, but otherwise comparable items (irrelevants). Previous work has shown that physical countermeasures can artificially modulate neural responses in concealed information tests, decreasing the accuracy of these methods. However, the question remains as to whether purely mental countermeasures, which are much more difficult to detect than physical ones, can also be effective. An fMRI study was conducted to address this question by assessing the effect of attentional countermeasures on the accuracy of the classification between knowledge and no‐knowledge cases using both univariate and multivariate analyses. Results replicate previous work and show reliable group activation differences between the probe and the irrelevants in fronto-parietal networks. Critically, classification accuracy was generally reduced by the mental countermeasures, but only significantly so with region of interest analyses (both univariate and multivariate). For whole‐brain analyses, classification accuracy was relatively low, but it was not significantly reduced by the countermeasures. These results indicate that mental countermeasure need to be addressed before these paradigms can be used in applied settings and that methods to defeat countermeasures, or at least to detect their use, need to be developed.