Algorithms May Be Picking Up Racial and Gender Bias as They Mirror Humans
Not great news for creating fairer loan and job applications.
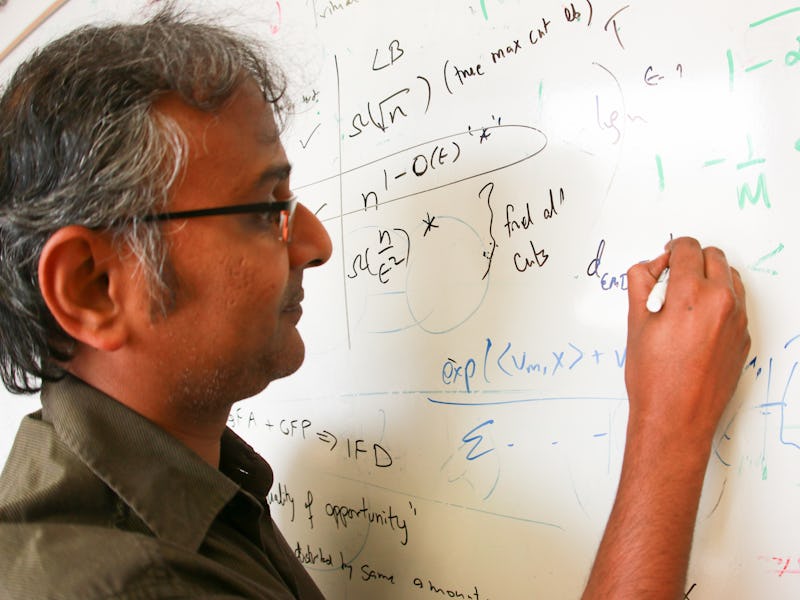
People who hire and give out loans have a pernicious set of biases. A person with a name perceived as black-sounding has to send out an average of 15 resumes to get the same number of responses as a white-sounding name gets in just 10. And black Americans were 50 percent more likely to have overpaid for mortgages from 2004 to 2008. Ideally, software should overcome the implicit and explicit discrimination that financial gatekeepers exhibit by cooly weighing an applicant’s merits. But computer scientists are finding that digital processes can be just as prejudiced, because guess who builds software? Yup: fallible ol’ humans.
A University of Utah research team lead by Suresh Venkatasubramanian has designed a test to determine whether an algorithm discriminates. The test uses the legal concept of “disparate impact,” which finds a policy is biased if it adversely effects a group based on any legally protected status, such as race or religion. Venkatasubramanian stops short of saying machine-learning algorithms are definitely spitting out biased results, but the potential to interpret a person’s race or gender in the data and discriminate accordingly is definitely there.
“The irony is that the more we design artificial intelligence technology that successfully mimics humans, the more that A.I. is learning in a way that we do, with all of our biases and limitations,” Venkatasubramanian told the University of Utah’s news service. “It would be ambitious and wonderful if what we did directly fed into better ways of doing hiring practices. But right now it’s a proof of concept.”
The good news here is that if we can determine how algorithms are discriminating, we can teach them how not to discriminate. And that’s a much easier talk than the one you might have had with that uncle who’s always posting Breitbart links on Facebook.