The Next Ebola Outbreak Is Hard to Predict, but "Outbreak Forecasting" Can Help
Disease surveillance remains one of the highest-stakes areas of science.
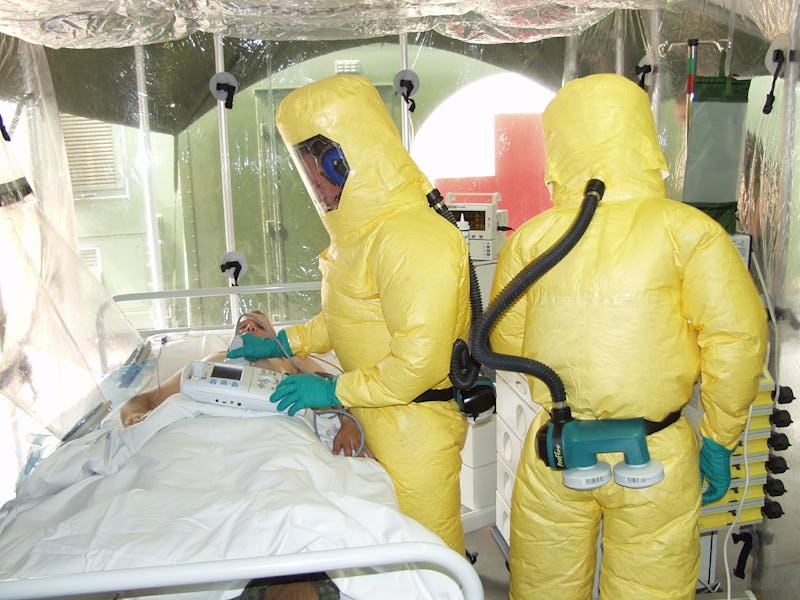
A 2-year-old boy in rural Guinea died of Ebola in December 2014. Over the next two years, almost 30,000 people in West Africa would be infected with the Ebola virus.
Why, unlike the previous 17 Ebola outbreaks, did this one grow so large, so quickly? What, if anything, can be done to prevent future outbreaks? These questions, along with many others, are at the heart of the nascent scientific field of outbreak forecasting. And the stakes couldn’t be higher. In January, the World Economic Forum called pandemics one of the greatest risks to business and human life.
Over the last several centuries, scientists have become ever better at predicting many aspects of the world, including the orbit of planets, the ebb and flow of tides, and the paths of hurricanes. The ability to understand natural and physical systems well enough to make accurate forecasts is perhaps one of humanity’s greatest achievements.
Much of this success at forecasting begins with Isaac Newton’s fundamental insight that there are unchanging universal laws that govern the natural phenomena around us. The ability to rapidly perform large calculations has fostered the Newtonian perspective that, given enough data and computing power, most complex phenomena can be predicted.
There are, however, limits. As scientists who study these kinds of predictive systems, we doubt that it will be possible to predict exactly what will happen next in a disease outbreak, because the most important variables can change so much from one outbreak to another.
This is why, as with weather forecasting, gathering real-time data is likely essential for advancing the scientific community’s ability to predict outbreaks.
Capricious Epidemics
The idea that scientists can model epidemics is based on the notion that the trajectory of each outbreak is predictable because of its intrinsic and unchanging properties.
Say a disease is caused by a transmissible pathogen. The infectiousness of that disease can be encapsulated in a number called the “basic reproductive ratio,” or R0, a number describing how widely a pathogen is likely to spread in a given population.
If epidemiologists know enough about a pathogen’s R0, the hope is that they can predict aspects of its next outbreak — and hopefully prevent small-scale outbreaks from becoming large-scale epidemics. They might do this by mobilizing resources to areas where pathogens have especially high R0 values. Or they might limit interactions between the carriers of disease and the most susceptible members of a given society, often children and the elderly.
In this way, R0 is interpreted as an immutable number. But modern studies demonstrate that this not the case.
For example, consider the Zika virus epidemic. For this disease, R0 ranged from 0.5 to 6.3. This is a remarkable span, ranging from a disease that will dissipate on its own to one that will cause a long-term epidemic.
One might think that this broad range of R0 values for Zika stems from statistical uncertainty — that maybe scientists just need more data. But that would be mostly incorrect. For Zika, myriad factors, from climate and mosquitoes to the presence of other related viruses like Dengue and the role of sexual transmission, all lead to different R0 values in different settings.
It turns out that the features of an epidemic — the pathogen’s contagiousness, rate of transmission, availability of vaccines, and so on — change so rapidly during the course of a single outbreak that scientists are able to predict dynamics only within the course of that outbreak. In other words, studying the Ebola virus disease outbreak in April 2014 may help scientists to understand an Ebola outbreak in that same setting the next month, but it’s often much less helpful for understanding the dynamics of future Ebola epidemics, such as the one that happened in May 2018.
Epidemics often aren’t neat and bundled phenomena. They are noisy occurrences where many variables play essential, but shifting, roles. There is no underlying truth of the disease — only an unstable collection of details that vary, often becoming entangled, as the disease spreads.
Better Predictions
If scientists aren’t confident they can understand epidemiological systems well enough to predict the behavior of related ones, why bother studying them?
The answer might reside in what we call a “soft physics” of prediction: Scientists should stop assuming that every outbreak follows the same rules. When comparing one outbreak with another, they should keep in mind all of the contextual differences between them.
The H1N1 influenza virus.
For example, biologists have uncovered many details about influenza infections. They know how the viruses bind to host cells, how they replicate, and how they evolve resistance to antiviral drugs. But one epidemic might have started when a large population used public transportation on a certain day of the month, while another might have been initiated by a congregation at a religious service. Though both outbreaks are rooted in the same infectious agent, these and many other differences in their particulars mean that scientists may need to reframe how they model how each progresses.
To understand these particulars better, scientists need significant investments in real-time data. Consider that the National Weather Service spends over $1 billion per year gathering data and making forecasts. The CDC spends only a quarter as much on public health statistics and has no dedicated budget for forecasting.
Disease surveillance remains one of the highest-stakes areas of science. A careful consideration for unique circumstances underlying outbreaks and more responsible collection of data could save thousands of lives.
This article was originally published on The Conversation by C. Brandon Ogbunu, Randall Harp, and Samuel V. Scarpino. Read the original article here.