Misinterpretation of Climate Data Comes Down to Political Loyalty
If you turn down the partisanship, you turn up the public understanding.
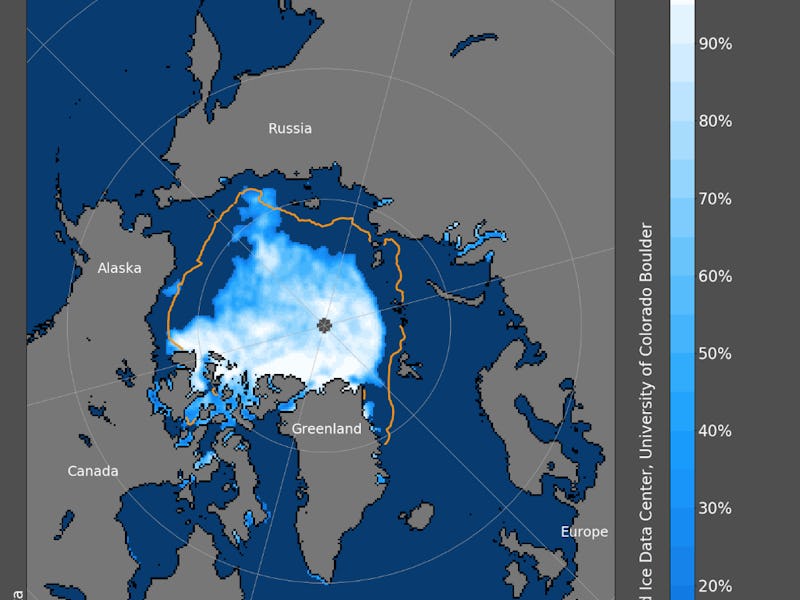
The amount of arctic sea ice around the North Pole has long been on a downward trend, and satellite data from the National Snow & Ice Data Center show this decline — particularly stark this time of year — with daily updates.
But there are spikes in any downward trend, and one particular spike in 2013 (thanks to an unusually cool summer) caused such widespread misinterpretation along political lines that it became the subject of a newly published sociological study. The findings show that when one strips away political affiliation, people make smarter decisions about climate science together. It also offers the latest scientific example of how politics don’t often allow facts to get in the way.
First, here’s the chart that researchers saw as problematic — sort of a political Rorschach test — that shows a spike in arctic sea ice in 2013. For instance, people who think climate change is a liberal hoax could point to the rise in sea ice as evidence the downward trend would soon return upward.
"The graph has been found to produce misinterpretations about the scientific information it communicates because its final data point indicates an increase in the amount of Arctic Sea ice in the opposite direction of the overall trend that NASA intended to communicate," write the researchers.
Damon Centola, a sociologist and professor at the Annenberg School for Communication at the University of Pennsylvania, led a study into why people might misinterpret the data above. His research team used social learning processes (showing answers from the rest of a group of people alongside the question) to see if they could eliminate polarization between self-identified Democrats and Republicans.
The research, “Social learning and partisan bias in the interpretation of climate trends,” was published Monday in the journal Proceedings of the National Academy of Sciences.
The sharpest baseline finding before the experiment began was that “Republicans significantly misinterpreted the data,” Centola says. “Overall, in every case, almost 40.2 percent of Republicans said that the arctic sea ice was increasing.” Meanwhile, 73.9 percent of liberals correctly estimated the sea ice trend at the baseline.
Centola, the senior author on the paper, and his team recruited 2,400 people, half Republican and half Democrat, on Amazon’s Mechanical Turk (the shipping giant’s “marketplace for work that requires human intelligence”). They were randomly assigned to 40-person bipartisan social networks to take an “intelligence test” that asked participants to forecast sea ice levels.
“The more accurate your answers, the more you win!,” the subjects of this study were informed. They were not informed that the data was determined by NASA, in order to avoid known biases associated with the organizational sources of information, write the researchers.
They were allowed to revise their responses while being show the responses of other people in their network, and when there was no party affiliation next to the responses of their network neighbors, their sea ice prediction were closer to the scientific prediction by NASA.
Other questions included symbols next to them, subtle suggestions that these science questions also had political gravity. When “subjects were exposed to party logos during communication, social learning was prevented, and baseline levels of polarization were maintained,” they write.
Just by placing the political symbols next to the data, the subjects provided different predictions, suggesting they were motivated by biased reasoning.
When all participants were presented with the data, asked to forecast based on that data, and informed they would be paid more money for accurate answers, Centola says the group “solved NASA’s problem” of people misinterpreting its research.
“Eight-five percent of both Republicans and Democrats agreed that arctic sea ice levels were in fact going down,” he says of the data that was presented nakedly, without affiliation or imagery. “And more importantly, the consensus was a much more accurate consensus for both groups.”
Four question types given to the study subjects. Researchers found that accuracy in sea ice forecasting increased when there were no partisan cues.
But when the data was presented with Republican elephant or Democrat donkey, or the words “conservative” or “liberal,” or a chart showing how people who identified as conservative or liberal voted, the forecasts skewed away from the correct results.
The charts show the change in accuracy of the four different types of questions after rounds 1 and 3, showing that "partisan priming," or the inclusion of political cues, hurt social learning.
“The benefits of social learning were not limited to conservatives,” the researchers write. “Liberals also improved in networks without partisan cues, finishing with significantly higher trend accuracy than liberals in the control condition. By the end of the study, in bipartisan networks without partisan cues, there were no longer significant differences in trend accuracy between the liberals and conservatives.”
When presented with the consensus from the group without party affiliation, the study subjects worked together to make the correct prediction.
“We find that in the absence of political imagery, cross-party contact eliminates polarization and leads to much better understanding of climate change,” Centola says.
Abstract
Vital scientific communications are frequently misinterpreted by the lay public as a result of motivated reasoning, where people misconstrue data to fit their political and psychological biases. In the case of climate change, some people have been found to systematically misinterpret climate data in ways that conflict with the intended message of climate scientists. While prior studies have attempted to reduce motivated reasoning through bipartisan communication networks, these networks have also been found to exacerbate bias. Popular theories hold that bipartisan networks amplify bias by exposing people to opposing beliefs. These theories are in tension with collective intelligence research, which shows that exchanging beliefs in social networks can facilitate social learning, thereby improving individual and group judgments. However, prior experiments in collective intelligence have relied almost exclusively on neutral questions that do not engage motivated reasoning. Using Amazon’s Mechanical Turk, we conducted an online experiment to test how bipartisan social networks can influence subjects’ interpretation of climate communications from NASA. Here, we show that exposure to opposing beliefs in structured bi- partisan social networks substantially improved the accuracy of judgments among both conservatives and liberals, eliminating belief polarization. However, we also find that social learning can be reduced, and belief polarization maintained, as a result of partisan priming. We find that increasing the salience of partisanship during communication, both through exposure to the logos of political par- ties and through exposure to the political identities of network peers, can significantly reduce social learning.