Google DeepMind's Mass Adoption is Being Held Back By This Flaw
As usual, it's YouTube to the rescue.
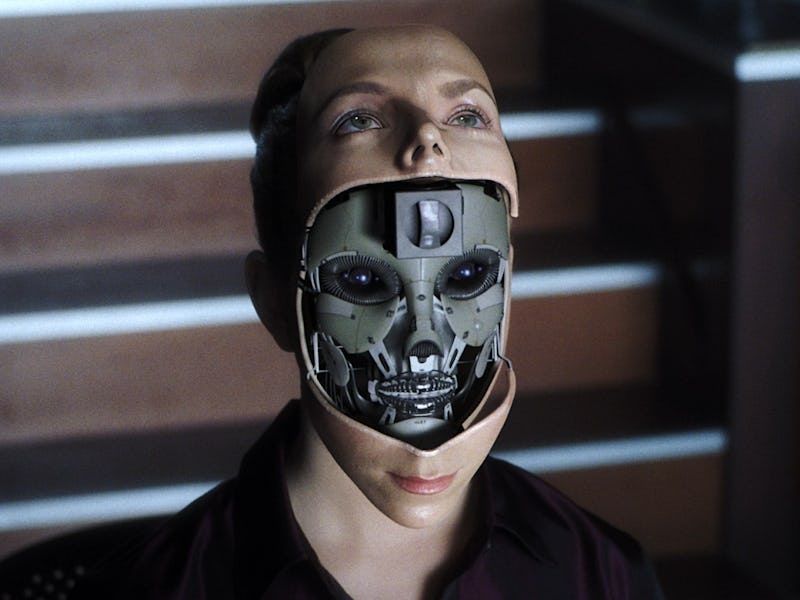
Google DeepMind is one of the world’s best A.I. development teams one need look no further than its latest success, AlphaGo’s victory over the world Go champion. The gold standard for A.I. has its limits, though, as revealed in a new paper.
Essentially, DeepMind’s programs might be able to tell a hawk from a handsaw, but ask it to tell a hand-wave from a hand-job, and you might not be so fortunate.
In a new, unpublished paper uploaded to the arXiv online repository, DeepMind researchers describe the creation of a new dataset designed to help A.I. programs learn how to identify different types of human actions and differentiate between them. The results indicate while A.I. can pretty accurately identify objects and individuals, they have an incredibly long way to go before they’ll be able to tell what those objects or people are actually doing.
The types of A.I. built by DeepMind and their rivals at places like Facebook and Apple use deep-learning algorithms which are taught to find patterns across insane troves of data. For example, in teaching a facial recognition program how to identify people and describe their emotions as perceived through faces, an A.I. would be introduced to hundreds of thousands or even millions of photographs of people and scan for clues that pinpoint what features most distinguish faces from one another, and what features and facial contortions best correlate with what kind of emotional states.
But recognizing static objects is one thing. Recognizing what a moving object is doing? In motion? That’s an entirely different thing — the addition of a z-axis on data that already possesses the x- and the y-axes. And for that, you need to show A.I. programs videos. Lots of them.
“A.I. systems are now very good at recognizing objects in images, but still have trouble making sense of videos,” a DeepMind spokesperson told Inverse. “One of the main reasons for this is that the research community has so far lacked a large, high-quality video dataset. We hope that the Kinetics dataset will help the machine learning community to advance models for video understanding, making a whole range of new research opportunities possible.”
The applications borne out of an A.I. program which can recognize and identify human action are enormous. Let’s say national security personnel is using A.I.-infused surveillance to scan for threats in a large crowd. Currently, a program might only be able to pick out suspects who have already been identified as potential threats. But a system which can recognize actions might be trained to flag down individuals who are doing suspicious things, like walking around in strange patterns, exhibiting unusual movements characteristic of suspects, and more. Beyond that, recognizing action might also pinpoint small details in, say, a beating heart, which are traits associated with arrhythmia or other disorders.
So the DeepMind team collated a collation of 300,000 video clips from YouTube which illustrate over 400 different classes of human actions. This Kinetics dataset dwarfs similar collections being used by the research community — primarily because Google is able to harness the sheer amount of video clips uploaded to YouTube (which Google owns).
The new paper illustrates how DeepMind A.I. systems trained off the Kinetics dataset exhibit an 80 percent or higher accuracy in classifying actions like “riding a mechanical bull,” “presenting weather forecast,” “sled dog racing,” “bowling,” and “picking fruit.”
List of 20 easiest and 20 hardest Kinetics classes sorted by class accuracies by DeepMind A.I.
Meanwhile, accuracies for classifying other actions like “tossing coin,” “shooting basketball,” “drinking beer,” “sneezing,” and slapping,” drop down to less than 20 percent for the DeepMind A.I.
Why? There are a ton of different reasons, but it usually just comes down to the fact that some actions are harder to identify without stronger, more vivid context clues. “Currently, much video understanding relies heavily on image understanding and is not able to reliably recognize dynamic patterns; for example, distinguishing different types of swimming or dancing,” said DeepMind’s spokesperson.
Moreover, training A.I. to recognize action patterns is still really new. The Kinetics dataset is one of the first robust training materials curated specifically for this cause. “The success in image understanding has been due to the use of neural network models, trained using deep learning,” says DeepMind. “However, these models require very large-scale datasets for their training. Such datasets are available for images, but until now, there have been no datasets of comparable size and quality available for videos.”
There is some hopeful news, however. The results indicate that the A.I. didn’t develop any gender-based biases in identifying action classes — meaning no single gender dominated the ID of actions. (There was, for obvious reasons, a gender imbalance when it came to certain actions like “shaving beard” — mostly male — and “cheerleading” — mostly female.) In the sense, DeepMind is making good progress in combating biases that have been a headache for A.I. researchers across the world.
If Google is serious about turning itself into an “A.I.-first” company, it’s certainly going about it better than its rivals.