NPR's Fascinating Plan to Use A.I. on Trump's Tweets
Can artificial intelligence read a tweet's sentiment?
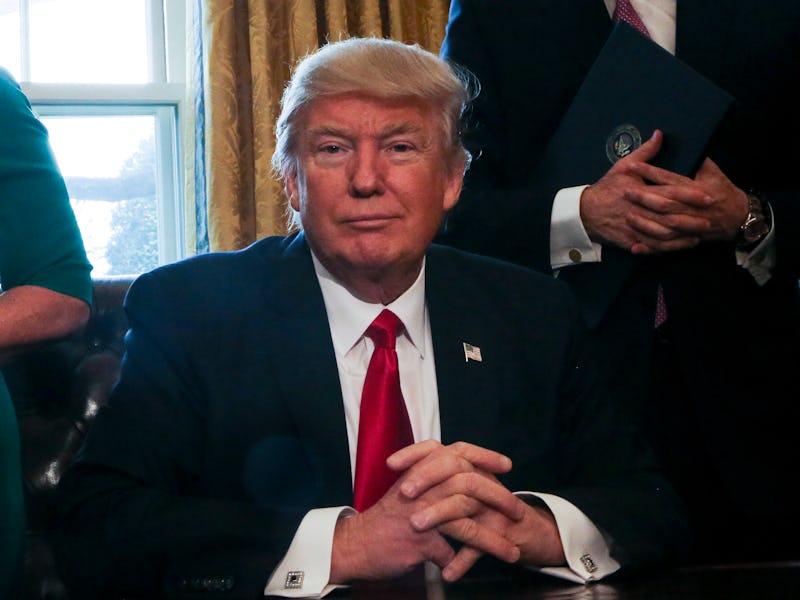
November 8, 2016 was a memorably tumultuous night for both now-President Donald Trump and Hillary Clinton, but it was also one in which the stock market showcased how sensitive it was to surprises and the political temperature of the country by nosediving dramatically.
Since then, the market has recovered. But on Monday, a simple artificial intelligence program — a bot — began tracking Trump’s personal Twitter account to see if the president’s tweets are a reliable predictor of stock prices in the American economy.
NPR’s Planet Money — the organization’s bi-weekly economics podcast — wondered if it could take Trump’s volatile tweeting and use it to its advantage to make investment decisions.
“We’re doing this all to learn how bots work,” Planet Money producer Alex Goldmark tells Inverse. “I figured it would be complicated and I think I was right.”
Goldmark’s bot, cutely named @BOTUS (after @POTUS), is an experiment that looks at how bots work outside of straightforward binary “yes” or “no” decisions, and how artificial intelligence interprets emotional intent, called “sentiment analysis” within the algorithm community.
Goldmark’s experiment isn’t without obstacles. There’s the financial, of course: Goldmark raised $1,000 of personal money from within the office. As one potential donor says with incredulity, “Wait, you really need 100 bucks from me? That’s not going to happen. I pay rent in New York. I’m not giving you $100.” But Goldmark insists that there’s a unique behavioral incentive to throwing in $100 precious bucks into the bucket — losing real money, after all, hurts. “I had to set up a real trading account and I paid a lot of attention to this,” he says. “The connections I made to use the bot, the fees I had to pay — all of that would be easy to ignore [if I hadn’t built it].”
Then there was the matter of programming this investment bot.
On the surface, the bot’s job is simple: If Trump tweets something positive about a company, the bot takes it as a sign of a potentially good investment; if Trump tweets something negative about a company, it’s a sign that the stock price for the product is going to take a tumble and that the Planet Money team will need to sell.
Scouring financial journalism articles helps with programming the bot to recognize not only the sentiment of the tweet but also context, like CEO names, product names, and brands. “If there is a tweet from @realdonaldtrump, [@BOTUS] provides a sentiment analysis from -1 to 1, or negative to positive, using a sentiment analysis algorithm,” Goldmark explains. “Then it looks to see if a company is mentioned. If a company is mentioned, it buys or sells stock. If it’s positive, the bot buys stock.” If it’s negative, the company sells.
But the simplicity of bots can work against them as well, and Goldmark consults with Tradeworx, a firm that builds bots in concert with traders, to understand how to best build a bot that can predict when to buy and sell based on tweets (Planet Money uses VADER Sentiment Analysis, available on Github). It’s a lot harder than it seems.
As the company’s head of investment, Mani Mahjouri, tells the podcast, “This is more than just positive and negative words. This is a computer actually determining the sentiment of a tweet. You can type any sentence in and it will give you a sense of what the sentiment was.”
Determining sentiment is way harder than it sounds. In a 2010 analysis of Twitter and sentiment analysis, researchers attempted to put together a method for bots to understand the sentiment of a tweet and realized that emotional text must be made explicit for bots to be able to understand sentiment. In other words, puns, sarcasm, double-entendres, and any other duplicitous form of speech will undermine sentiment analysis; straight talk’s the way, at least so far.
Which, if you think about it, is odd, given how much sarcasm thrives on Twitter and how downright mean Siri and other similar voice-activated artificial intelligence can be. Bots are starting to pick up on sarcasm, though, as a 2016 study shows, though history and context are necessary for the bot to figure out that a person is being disingenuous. And there’s reason to believe the great political speeches of the future will be written by robots.
Luckily, Trump is a literal sort of Twitter user, an analysis of @realdonaldtrump’s tweets show. “If you look at Donald Trump’s Twitter feed and sentiment scores, they’re either very positive or very negative,” Goldmark points out. “There are very few that are in the middle or neutral.” The one surprising “neutral” ground in Trump’s tweets were retweets of newspaper headlines — which Goldmark says makes sense, given the fact that headlines are neutral, though Trump may play the headline to his political agenda. “Headlines are a statement of fact,” Goldmark says. “You won’t see something like Awesome Ford Plant to Open in Amazing Michigan, for example.” (Fun fact: Saturday Night Live got a ton of negative sentiments.)
Though @BOTUS was launched on Friday afternoon, Monday was the first day of business for the bot; as of publication, @BOTUS hasn’t made any trades — but that’s because the president hasn’t tweeted anything from his personal Twitter handle one way or the other — yet. There’s no end time to the project — “We’ll go as long as it’s interesting,” Goldmark says — and Goldmark is pretty sure their small investment will have pretty slim returns, if any at all.
Is this the future of trading? Goldmark laughs. “We [the Planet Money team] are terrible investors,” he says. “Just about everything we’ve invested in has failed.” Who knows, though, how a bot might change things.