Is Your Doctor Stumped? There's a Chatbot for That
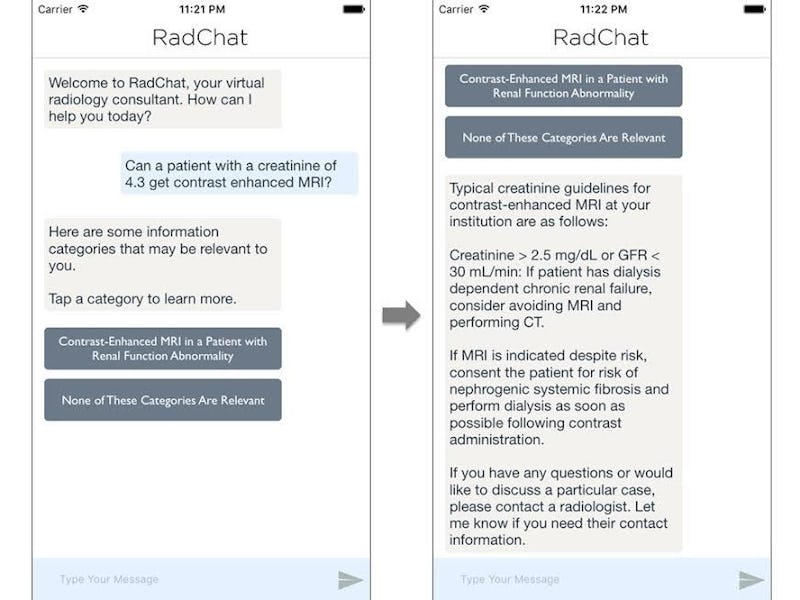
Doctors have created a chatbot to revolutionize communication within hospitals using artificial intelligence technology from self-driving cars. RadChat, which is basically a cyber-radiologist in app form, can quickly and accurately provide specialized information to non-radiologists. And, like all good A.I., it’s constantly learning.
Traditionally, interdepartmental communication in hospitals is a hassle. A clinician’s assistant or nurse practitioner with a radiology question would need to get a specialist on the phone, which can take time and risks miscommunication. But using the app, non-radiologists can plug in common technical questions and receive an accurate response instantly. A team of interventional radiologists at the University of California, Los Angeles, designed the chatbot via deep learning technology, which is to say technology modeled after the human brain. The research was presented Wednesday at the Society of Interventional Radiology’s Annual Scientific Meeting.
"RadChat" 👌
“Say a patient has a creatinine [lab test to see how well the kidneys are working]” co-author and application programmer Kevin Seals tells Inverse. “You send a message, like you’re texting with a human radiologist. ‘My patient is a 5.6, can they get a CT scan with contrast?’ A lot of this is pretty routine questions that are easily automated with software, but there’s no good tool for doing that now.”
Lest you get chills from the idea of someone announcing to a waiting room that “the chatbot will see you now,” know that the system doesn’t interface with patients directly. The goal is to improve patient care through cutting-edge technology, but it does that by making communication more efficient based on quantitative criteria. Healthcare, already in the throes of apps (like, a lot of apps) is widely perceived as one of the industries to benefit heavily from A.I. in the near future, and the UCLA team is here for that.
“It’s great because since all the recommendations built into the app were carefully compiled by expert rediologists, not only does the clinician get the information rapidly but it’s exceptionally high-quality, carefully vetted, and evidence-based,” Seals says. “And as each message and selected response gets stored, it’s building a really, really rich bank of data.”
As RadChat is fed more data and used in more scenarios, it’s growing smarter and smarter. This long, perfect record of communication allows the doctors to identify new functionality they can implement into the system in the future. The team designed the chatbot for radiology because they are radiologists themselves, but there’s no reason a cardiology chatbot or a neurosurgery chatbot, etc., couldn’t be built using the same framework; the underlying idea of aggregating traditionally siloed specialties for quick info-dissemination is the same.
Now that they know they have a fully functional technology that works smoothly, the next step is to test it with collaborators and get wider feedback. In about a month, the team plans to make the chatbot available to everyone at UCLA’s Ronald Reagan Medical Center, see how that plays out, and scale up from there. Your doctor may never be stumped again.
Abstract
Purpose: Improved artificial intelligence through deep learning has the potential to fundamentally transform our society, from automated image analysis to the creation of self-driving cars. We apply these techniques to create a virtual radiology assistant that offers clinicians many non-interpretive radiology skills. A wide range of functionality is offered, from helping select the optimal imaging study or procedure for a given clinical scenario to describing the follow-up of incidental findings, guiding contrast administration in renal failure/contrast allergy, and describing optimal peri-procedural patient management.
Materials: The application was built in Xcode using the Swift programming language. The user interface consists of text boxes arranged in a manner simulating communication via traditional SMS text messaging services. Natural Language Processing (NLP) was implemented using the Watson Natural Language Classifier application program interface (API). Using this classifier, user inputs are understood and paired with relevant information categories of interest to clinicians. For example, if a clinician asks whether IVC filter placement is appropriate for a particular patient, they will be paired with an IVC filter category and relevant information will be provided. This information can come in multiple forms, including relevant websites, infographics, and subprograms within the application.
Results: Model performance improves significantly as more training data is provided. Successful input categorization is performed in most cases, particularly for focused queries providing sufficient detail. Using a confidence probability threshold of 0.7, the natural language classifier provides optimal information categorization with minimal inclusion of extraneous categories.
Conclusions: Deep learning techniques can be used to create powerful artificial intelligence tools to assist clinicians. These tools both allow clinicians to rapidly access useful information and reduce the need for radiologists to perform redundant communication tasks that potentially distract from patient care.