This Mathematician's Oscar Prediction Model Is Almost Flawless
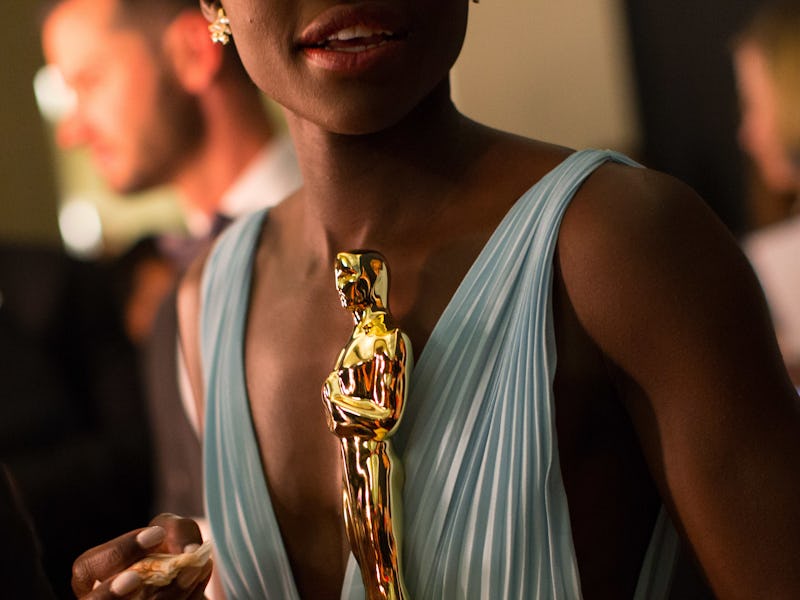
Since 2005, Iain Pardoe, Ph.D., has gotten over 75 percent of his Oscar predictions right — and he’s getting more accurate each year. The statistical consultant’s computer model analyzes swaths of data relating to all Oscar winners since 1928, allowing him to determine which factors are the most important in making predictions. Just in time for awards season, he shared his tips for winning an Oscars pool with Inverse.
His statistical model, described in an article published in Statistics In Society, focuses only on predicting the winners for the four major Oscar categories — Best Picture, Best Director, and Best Actor and Actress — because he simply didn’t have the time to sift through nearly a century’s worth of data for all of these categories. Most of it turned out to be irrelevant, anyway; for these categories, he says, “there are only a few factors that would help.”
Best Picture
“The overall number of nominations that a movie gets turns out to be important for Best Picture and Best Director but doesn’t have an impact on acting,” he says. This is corroborated by data from recent years: In 2015, for example, Birdman, directed by Alejandro G. Iñárritu, took home both Best Picture and Best Director awards. (2016’s Best Picture winner, Spotlight, he says, was a “surprise,” although its director Tom McCarthy was also nominated for Best Director.)
Best Director
Similarly, Best Director winners are best predicted by looking at whether the film associated with the director has been nominated for Best Picture. “I think it’s only happened a few times that a movie has won and the director wasn’t nominated,” he says.
He points out that the Best Director category has “become more interesting” in the last couple of years because it involves fewer nominations than Best Picture (it involves only five nominees, while Best Picture has varied between 5 and 10 since 2011).
The number of Oscar nominations an actor has had in the past is a big factor in whether he will take home the award. The same doesn't go for actresses, though.
Best Actor and Actress
For both of these categories, the number of times the actor or actress has won before makes a big difference. “It’s actually very difficult for an actor or actress to win more than once,” he says.
But it’s when considering the number of nominations an actor or actor has had that the trends become unusual. The number of times a person has been nominated before is “important” for actors, he says — he points to Denzel Washington’s win over crowd favorite Russell Crowe in 2011, saying “it was due, I think” — but when it comes to actresses, it doesn’t make a difference.
General Tips
“For all four categories, the Guild Awards matter,” he says, referring to the prizes handed out by SAG-AFTRA each year before the Oscars. “I’m always surprised that people don’t make more of the Guild Awards.”
In addition, his statistical model has also shown that, of all of the other critics’ awards handed out annually, the one that is most predictive is the Golden Globes.
Take note of the Guild Award nominees for 2017.
In 2008, 2009, 2013, and 2014, Pardoe’s model got all of its predictions correct. Getting two out of four right, in 2011, was “the worst I’ve done in the past 11 years,” he says. Each year, he updates his model with the relevant data and runs the analysis again; there is so much number-crunching involved that it usually has to run overnight.
For statisticians, however, it’s the anomalies that are the most curious. “You can look all the way back to 1938, and look at where the surprises were,” he says. Nominees that have a high predicted probability of winning that lose to unexpected picks — he recalls Million Dollar Baby’s win over The Aviator in 2004 — are, to him, “more interesting.”
Despite his previous Oscar success — Pardoe admits to having won a set of Best Picture-nominated DVDs from an online pool — he won’t take responsibility for anyone’s wins or losses if they use his model’s predictions. “It tends to do pretty well, but not flawlessly,” he says. He’s still waiting on the Guild Award winners to be announced on January 29 before running his model for this year’s picks, but he’ll be posting them on his website as soon as they’re predicted.