Facebook Is Teaching A.I. How to See
A trinity of neural nets that could revolutionize everything from image search to autonomous car technology.
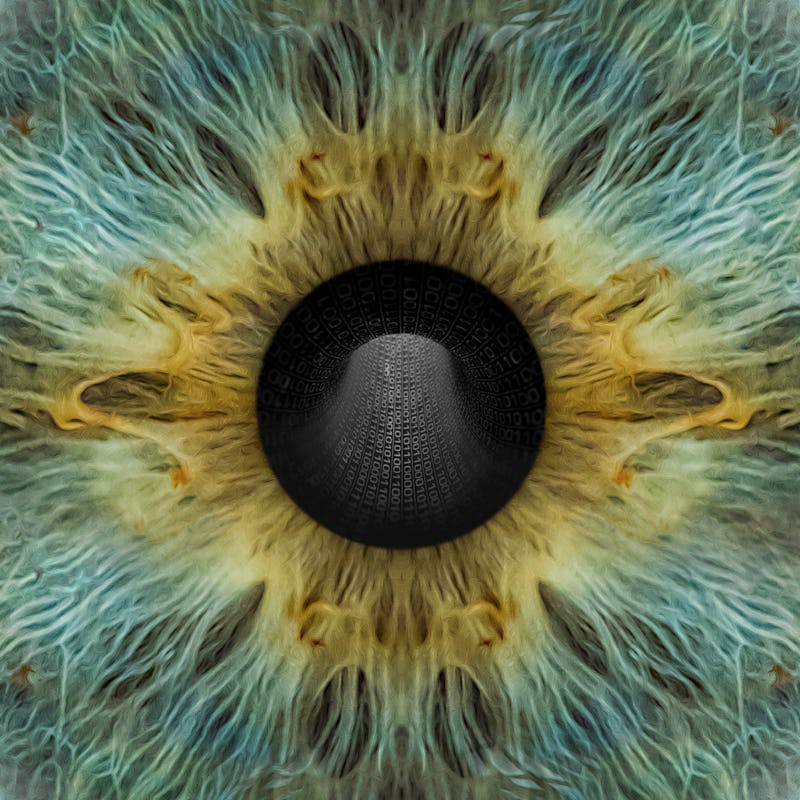
On Thursday, Facebook announced another gift to the artificial intelligence community when it made its computer vision tools open-source, with hopes that one day computers will be able to recognize images and relay that information to people who can’t see.
It comes down to three visual elements — DeepMask, SharpMask, and MultiPathNet — that comprise a powerful tool designed to bestow the wonder of sight upon now-blind computers. And in turn, those computers will help bestow sight to blind humans, improve image search, and could one day even help make cars fully autonomous.
If we’re lucky, we come into the world with functioning eyes. Soon enough, we begin to understand what we’re seeing, then we begin to understand that we’re seeing things. After a bit more time passes, we come to know these things as objects, and learn what to call them.
Most A.I. systems today know that there’s a photo or a video, and that’s it. With advanced A.I., computers can understand that a photograph contains faces, or a landscape. Beyond that, even the most capable A.I.s struggle to isolate objects in photographs, let alone identify them. A photograph, to a computer, is just a hodgepodge of data (though these data contain more information than you might assume).
Before and after a photo is "encoded as an array of numbers representing color values for each pixel."
Enter Facebook A.I. Research Scientist Piotr Dollár, with his A.I trinity: DeepMask, SharpMask, and MultiPathNet. DeepMask is like a pair of undeveloped eyes with no brain attached. Everything is pretty blurry and ill-defined, and DeepMask, lacking a brain, cannot describe the various blobs it sees. SharpMask, though, is like a pair of prescription glasses. Give these glasses to DeepMask and everything comes into focus. Still, the duo cannot describe what they see. This is where MultiPathNet, the brain, comes in. It looks at what DeepMask and SharpMask have together seen, and, because it’s been instructed well, identifies the now-clear and well-defined objects.
In less metaphorical terms: DeepMask takes an image and begins to segment its constituents. It’s not extremely adept at doing so: Objects are not well-delineated. SharpMask uses these results and employs its own method, tightening up the various objects’ boundaries until they’re clear-cut. If a photo contains a monkey, a beer, and a switchblade, DeepMask and SharpMask will spit out an image with one highlighted monkey shape, one highlighted beer shape, and one highlighted switchblade shape. MultiPathNet takes this image, with its accurate segmentation, and analyzes what the shapes are. It can identify the monkey shape as a “monkey,” and do the same for whatever other segmented objects appear in the image.
Quality image segmentation has long eluded computer scientists.
This may not seem altogether revolutionary. And for now, while it is a major advance for A.I., it’s unlikely to have a noticeable effect on your Facebook or internet browsing experience. But — especially because it’s now open-source — the trinity will only continue to improve. When it’s full-grown, your browsing experience will be noticeably better, and hitherto unimagined applications will arise.
Facebook’s current A.I. can tell blind people what an image “may contain,” which enhances their experience on the site and is less exclusionary. As this system matures, the descriptions will grow more and more accurate, and the experiences richer and richer. “Our goal is to enable even more immersive experiences that allow users to ‘see’ a photo by swiping their finger across an image and having the system describe the content they’re touching,” Dollár writes.
Various images fed through the A.I. trinity, segmented, and captioned.
But sighted Facebookers will benefit, too. Image search will improve, as Facebook will know what’s in each image regardless of its caption and tags. Other developers will soon incorporate these computer vision A.I.s: In one imagined scenario, an app will scan a photo of, say, a bagel sandwich, and — with the sandwich’s various ingredients segmented and identified (ham, cheese, egg) — estimate the nutritional content in said bagel sandwich.
Two imagined applications.
Another likely application will be self-driving cars, which rely on computer vision. Tesla Motors CEO Elon Musk has said that it’s no longer insufficient hardware holding fully autonomous cars back — it’s insufficient software. Computers, if they’re to drive for us, need to understand a car’s surroundings better than even we do. They need to be able to identify other cars, obstacles, signs, road markings, stoplights, pedestrians, bikers, animals — anything a driver might encounter on the road. Some autonomous car developers are crowdsourcing their A.I. instruction: If humans repeatedly show an A.I. where the cars and signs and so on are, then the A.I. will learn — albeit gradually — to pick out these objects itself. But with Facebook’s now open-source, tripartite computer vision system, these computers will comprehend their surroundings better, and sooner, than they otherwise would. As a result, self-driving cars would be far safer.
That may be some time off, but it’s at least in the pipeline. For now, static images are complicated enough. The next step is identifying and describing objects in videos, which are each day establishing a firmer grip on your Newsfeeds. Parsing and naming objects in live video is yet another daunting hurdle, which is part of what Musk and other self-driving car developers need to overcome for full autonomy.
Musk is concerned about A.I. in general, but a self-driving car’s “narrow” A.I. — which is A.I. that perfects only one task — is not something that worries him. On its own, teaching an A.I. to “see” is indeed narrow. But add an understanding of the image, and the slice grows yet larger. With this trinity, we’re teaching computers how to see; they already know how to hear and read, and it won’t be long before someone figures out how to combine these and other A.I.s into one beautiful beast.
In other words: After enough narrow A.I.s exist, the capacity to meld a broad A.I. skyrockets. Facebook is leading the charge. For now, given its researchers’ generosity with code and its impressive results, we should thank Facebook. For someone like Musk, whether our descendants will likewise show gratitude remains to be seen.