DARPA to Build "Virtual Data Scientist" Assistants Through A.I.
A.I. will make up for the lack of data scientists.
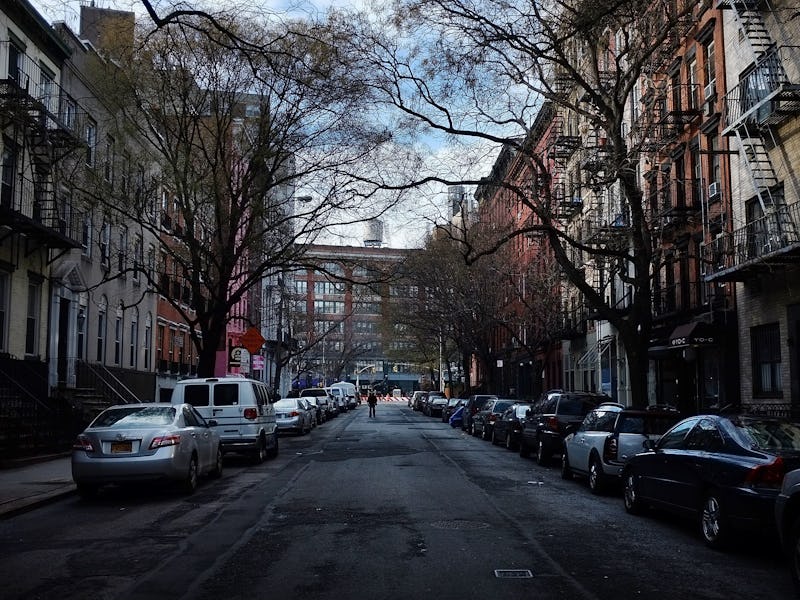
The Defense Advanced Research Projects Agency (DARPA) announced on Friday the launch of Data-Driven Discovery of Models (D3M), which aim to help non-experts bridge what it calls the “data-science expertise gap” by allowing artificial assistants to help people with machine learning. DARPA calls it a “virtual data scientist” assistant.
This software is doubly important because there’s a lack of data scientists right now and a greater demand than ever for more data-driven solutions. DARPA says experts project 2016 deficits of 140,000 to 190,000 data scientists worldwide, and increasing shortfalls in coming years.
For example, in order to construct a model for how different weather, school, location, and crime factors affect congestion for ride-sharing services in downtown Manhattan, a team of NYU students spent the equivalent of more than 90 months of work hours to complete the model. DARPA sees problems just like this all the time and the D3M Program will strive to construct it to drastically reduce the time and expertise needed to make models like these in the future.
“The construction of empirical models today is largely a manual process, requiring data experts to translate stochastic elements, such as weather and traffic, into models that engineers and scientists can then ask questions of,” said Wade Shen, program manager in DARPA’s Information Innovation Office. “We believe it’s possible to automate certain aspects of data science, and specifically to have machines learn from prior example how to construct new models.”
This flowchart illustrates how the D3M A.I. might work.
As a defense agency, of course DARPA is also looking into how this A.I. could affect the battlefield and save more lives.
Google is already using its A.I. to do similar tasks such as Alphabet’s Sidewalk Labs’ partnership with the U.S. Department of Transportation’s Smart City Challenge, which aims to use data-collecting infrastructure to help ease congestion and parking in vying cities.
If smaller teams of data scientists and non-experts can use machine learning models to help identify problems in society, there will be more time for analysis of the data to actually implement solutions.
“Our ability to understand everything from traffic to the behavior of hostile forces is increasingly possible given the growth in data from sensors and open sources,” said Shen. “The hope is that D3M will handle the basics of model development so people can apply their human intelligence to look at data in new ways, and imagine solutions and possibilities that were not obvious or even conceivable before.”