Weaponizing Machine Learning Against ISIS Will Tangle Military Chains of Command
Automation is neither good nor bad on its own, but it's worrisome in the Situation Room.
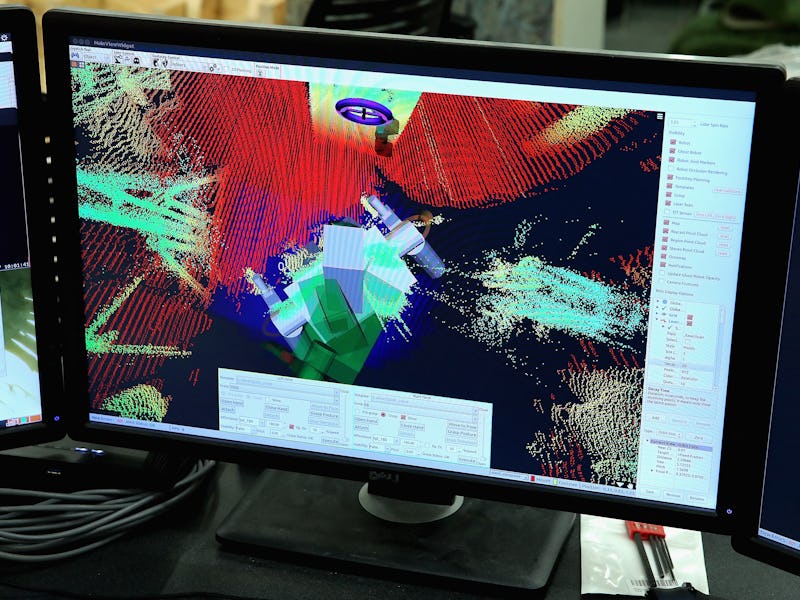
Everyone on the internet had a great time with Tay, Microsoft’s Twitter robot that became a racist Holocaust denier in a matter of a few hours (then came back and did it again). The company had created a public relations flap — more incident than a disaster — while giving the public an object lesson on the pros and cons of machine learning: Automation can harness patterns to fascinating effect at speed, but the results will be predictably hard to predict.
As is often the case, the military is an early adopter of automation technology. It is — at one time — leading the charge toward machine learning and also trying desperately to keep up. One of the main areas of focus for the Pentagon is autonomous robots and how they will team with humans – a R2D2-style robot wingman, for instance. But this week, Deputy Secretary of Defense Robert Work outlined another task for A.I.: open-source data crunching.
“We are absolutely certain that the use of deep-learning machines is going to allow us to have a better understanding of ISIL as a network and better understanding about how to target it precisely and lead to its defeat,” said Secretary Work, according to DoD’s website. According to that account, Work, who was speaking at an event organized by the Washington Post, had his epiphany while watching a Silicon Valley tech company demonstrate “a machine that took in data from Twitter, Instagram and many other public sources to show the July 2014 Malaysia Airlines Flight 17 shoot-down in real time.”
Private companies and law enforcement have been attempting to make sense of “big data” for a long time. But the military has an advantage: resource. Also, they’ve got access to classified materials.
The U.S. government seems ready to bet that software algorithms can sort through the massive amount of data out there in order to identify ISIS targets that would otherwise have eluded them, and detect and disrupt plots before the planners are able to carry them out. The government is already trying to study social media to predict the size of online protests. There’s no question that machine-learning will give intelligence analysts increasing power to make sense of the wealth of available information in the world. But when that intelligence becomes the basis upon which a lethal strike is taken, the ethical issues become more complex, even if they seem straightforward.
Though Work was quick to state that the Pentagon would not “delegate lethal authority to a machine,” that remains the end game. In the meantime, humans will remain “in the loop,” as the jargon goes. But as anyone who has looked at an iPhone for a weather report when standing next to a window knows, the relationships we have with our devices and software is not simple. We’re problematically credulous and easily distracted by UI issues.
“Automation bias,” the tendency for humans to defer to machines, presents a clear and increasingly present danger. The go-to example to illustrate this phenomenon is when your phone tells you to take a travel route you know is wrong but you do it anyway, presuming that the phone must know something you don’t. This is a common problem in non-military contexts. What the Pentagon appears to be stepping closer too, though, is threat reports composed by artificial intelligence. We don’t know anything about the potential efficacy of this program other than that it will be hard for humans to implement.
In a 2001 paper looking at student and professional pilots and automation bias, researchers found that “in scenarios in which correct information was available to cross check and detect automation anomalies, error rates approximating 55% were documented across both populations.” The study also found that adding an additional human teammate didn’t mitigate the problem.
Similarly, an MIT study from last year somewhat disturbingly found that computer and video game players had a “higher propensity to overtrust automation.” That could mean that the more time we spend staring at our screens, the more we trust what we see. Again, the problem isn’t with the systems we use, but with the way we use them. The fault is not in our stars, but in ourselves.
Big data remains promising. Machine learning remains promising. But when machines advise humans, results are predictably unpredictable. Does Tay’s transformation into a neo-Nazi misogynist mean that the Twitter hates Jews and women? It’s hard to know, but fairly unlikely. When we don’t understand the process by how inputs become outputs, we struggle to deal with the results in a rational way. Which puts the Pentagon in an interesting position. Are the people programming the military’s machine learning software going to be the ones to order airstrikes? That’s not how chain of command works, but chains of command get tangled when technology gets involved.